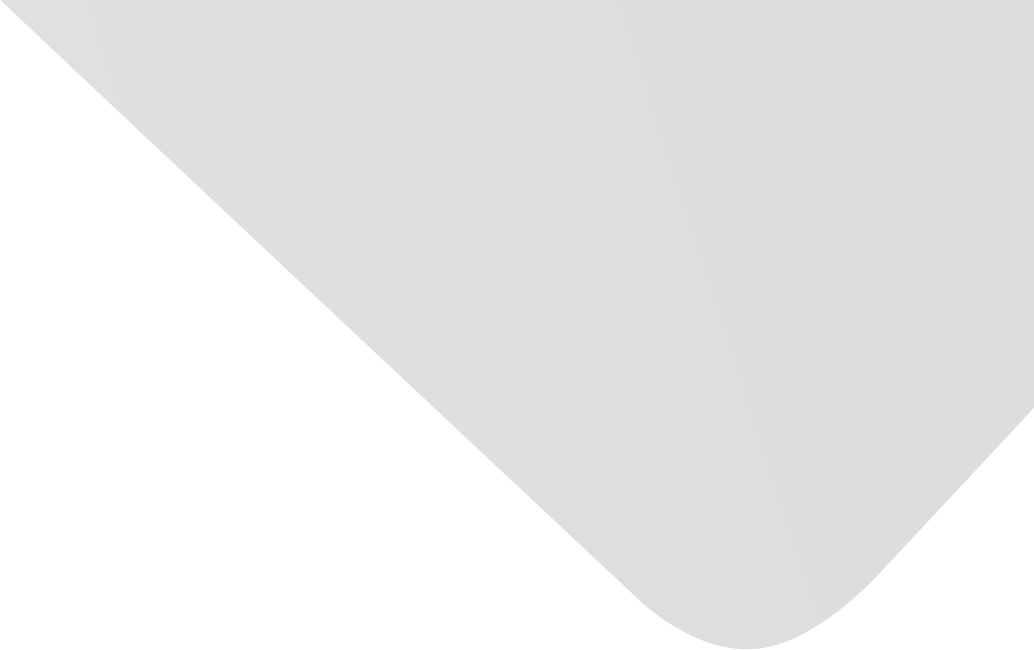
Improving Rolling Bearing Fault Diagnosis by DS Evidence Theory Based Fusion Model
Joint Authors
Yao, Xuemei
Li, Shaobo
Hu, Jianjun
Source
Issue
Vol. 2017, Issue 2017 (31 Dec. 2017), pp.1-14, 14 p.
Publisher
Hindawi Publishing Corporation
Publication Date
2017-10-22
Country of Publication
Egypt
No. of Pages
14
Main Subjects
Abstract EN
Rolling bearing plays an important role in rotating machinery and its working condition directly affects the equipment efficiency.
While dozens of methods have been proposed for real-time bearing fault diagnosis and monitoring, the fault classification accuracy of existing algorithms is still not satisfactory.
This work presents a novel algorithm fusion model based on principal component analysis and Dempster-Shafer evidence theory for rolling bearing fault diagnosis.
It combines the advantages of the learning vector quantization (LVQ) neural network model and the decision tree model.
Experiments under three different spinning bearing speeds and two different crack sizes show that our fusion model has better performance and higher accuracy than either of the base classification models for rolling bearing fault diagnosis, which is achieved via synergic prediction from both types of models.
American Psychological Association (APA)
Yao, Xuemei& Li, Shaobo& Hu, Jianjun. 2017. Improving Rolling Bearing Fault Diagnosis by DS Evidence Theory Based Fusion Model. Journal of Sensors،Vol. 2017, no. 2017, pp.1-14.
https://search.emarefa.net/detail/BIM-1187225
Modern Language Association (MLA)
Yao, Xuemei…[et al.]. Improving Rolling Bearing Fault Diagnosis by DS Evidence Theory Based Fusion Model. Journal of Sensors No. 2017 (2017), pp.1-14.
https://search.emarefa.net/detail/BIM-1187225
American Medical Association (AMA)
Yao, Xuemei& Li, Shaobo& Hu, Jianjun. Improving Rolling Bearing Fault Diagnosis by DS Evidence Theory Based Fusion Model. Journal of Sensors. 2017. Vol. 2017, no. 2017, pp.1-14.
https://search.emarefa.net/detail/BIM-1187225
Data Type
Journal Articles
Language
English
Notes
Includes bibliographical references
Record ID
BIM-1187225