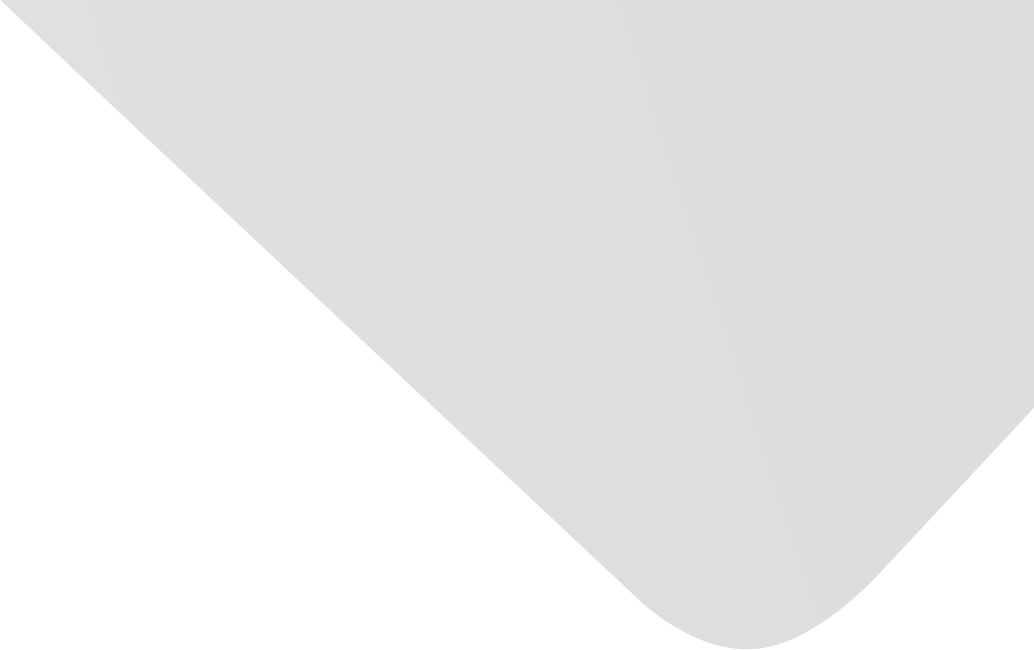
Visual Navigation with Asynchronous Proximal Policy Optimization in Artificial Agents
المؤلفون المشاركون
المصدر
العدد
المجلد 2020، العدد 2020 (31 ديسمبر/كانون الأول 2020)، ص ص. 1-7، 7ص.
الناشر
Hindawi Publishing Corporation
تاريخ النشر
2020-10-15
دولة النشر
مصر
عدد الصفحات
7
التخصصات الرئيسية
الملخص EN
Vanilla policy gradient methods suffer from high variance, leading to unstable policies during training, where the policy’s performance fluctuates drastically between iterations.
To address this issue, we analyze the policy optimization process of the navigation method based on deep reinforcement learning (DRL) that uses asynchronous gradient descent for optimization.
A variant navigation (asynchronous proximal policy optimization navigation, appoNav) is presented that can guarantee the policy monotonic improvement during the process of policy optimization.
Our experiments are tested in DeepMind Lab, and the experimental results show that the artificial agents with appoNav perform better than the compared algorithm.
نمط استشهاد جمعية علماء النفس الأمريكية (APA)
Zeng, Fanyu& Wang, Chen. 2020. Visual Navigation with Asynchronous Proximal Policy Optimization in Artificial Agents. Journal of Robotics،Vol. 2020, no. 2020, pp.1-7.
https://search.emarefa.net/detail/BIM-1190254
نمط استشهاد الجمعية الأمريكية للغات الحديثة (MLA)
Zeng, Fanyu& Wang, Chen. Visual Navigation with Asynchronous Proximal Policy Optimization in Artificial Agents. Journal of Robotics No. 2020 (2020), pp.1-7.
https://search.emarefa.net/detail/BIM-1190254
نمط استشهاد الجمعية الطبية الأمريكية (AMA)
Zeng, Fanyu& Wang, Chen. Visual Navigation with Asynchronous Proximal Policy Optimization in Artificial Agents. Journal of Robotics. 2020. Vol. 2020, no. 2020, pp.1-7.
https://search.emarefa.net/detail/BIM-1190254
نوع البيانات
مقالات
لغة النص
الإنجليزية
الملاحظات
Includes bibliographical references
رقم السجل
BIM-1190254
قاعدة معامل التأثير والاستشهادات المرجعية العربي "ارسيف Arcif"
أضخم قاعدة بيانات عربية للاستشهادات المرجعية للمجلات العلمية المحكمة الصادرة في العالم العربي
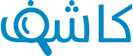
تقوم هذه الخدمة بالتحقق من التشابه أو الانتحال في الأبحاث والمقالات العلمية والأطروحات الجامعية والكتب والأبحاث باللغة العربية، وتحديد درجة التشابه أو أصالة الأعمال البحثية وحماية ملكيتها الفكرية. تعرف اكثر
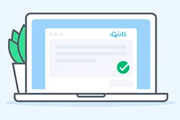