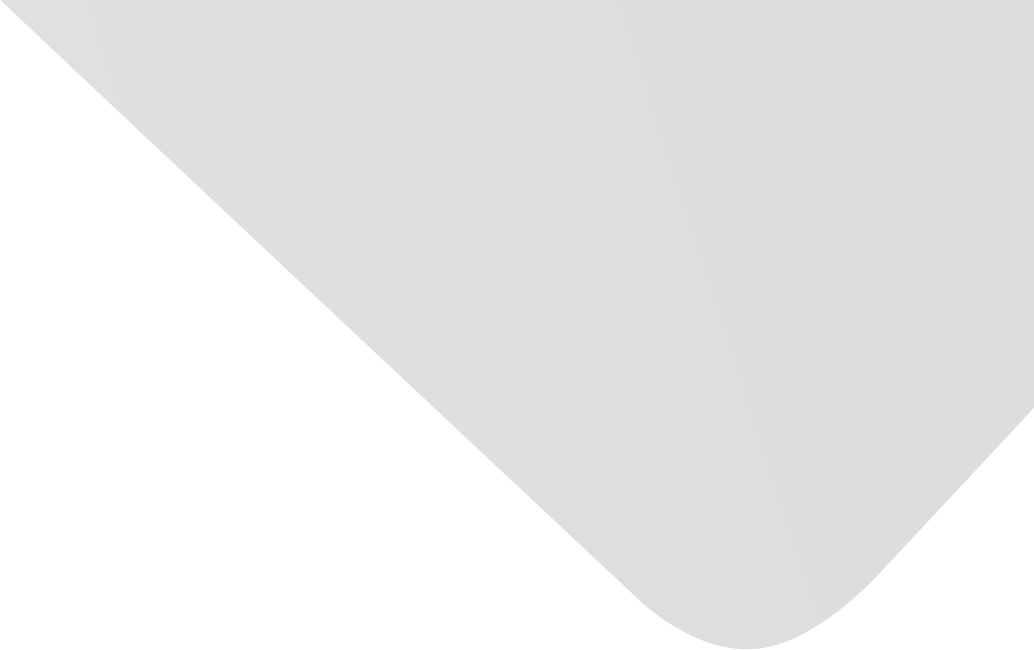
Visual Navigation with Asynchronous Proximal Policy Optimization in Artificial Agents
Joint Authors
Source
Issue
Vol. 2020, Issue 2020 (31 Dec. 2020), pp.1-7, 7 p.
Publisher
Hindawi Publishing Corporation
Publication Date
2020-10-15
Country of Publication
Egypt
No. of Pages
7
Main Subjects
Abstract EN
Vanilla policy gradient methods suffer from high variance, leading to unstable policies during training, where the policy’s performance fluctuates drastically between iterations.
To address this issue, we analyze the policy optimization process of the navigation method based on deep reinforcement learning (DRL) that uses asynchronous gradient descent for optimization.
A variant navigation (asynchronous proximal policy optimization navigation, appoNav) is presented that can guarantee the policy monotonic improvement during the process of policy optimization.
Our experiments are tested in DeepMind Lab, and the experimental results show that the artificial agents with appoNav perform better than the compared algorithm.
American Psychological Association (APA)
Zeng, Fanyu& Wang, Chen. 2020. Visual Navigation with Asynchronous Proximal Policy Optimization in Artificial Agents. Journal of Robotics،Vol. 2020, no. 2020, pp.1-7.
https://search.emarefa.net/detail/BIM-1190254
Modern Language Association (MLA)
Zeng, Fanyu& Wang, Chen. Visual Navigation with Asynchronous Proximal Policy Optimization in Artificial Agents. Journal of Robotics No. 2020 (2020), pp.1-7.
https://search.emarefa.net/detail/BIM-1190254
American Medical Association (AMA)
Zeng, Fanyu& Wang, Chen. Visual Navigation with Asynchronous Proximal Policy Optimization in Artificial Agents. Journal of Robotics. 2020. Vol. 2020, no. 2020, pp.1-7.
https://search.emarefa.net/detail/BIM-1190254
Data Type
Journal Articles
Language
English
Notes
Includes bibliographical references
Record ID
BIM-1190254