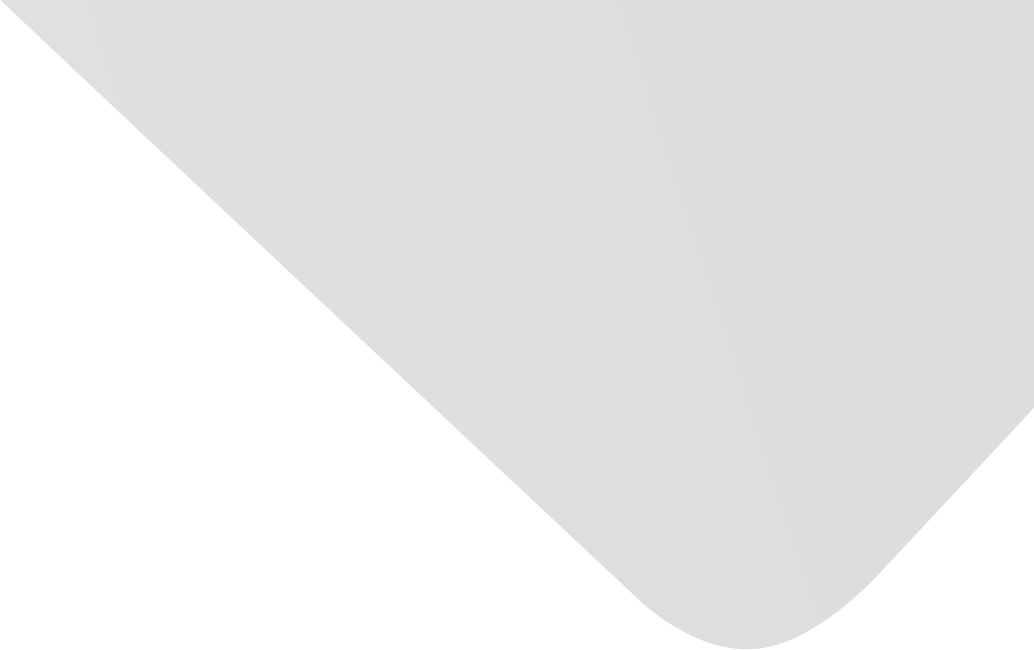
Intrusion Detection System for Internet of Things Based on Temporal Convolution Neural Network and Efficient Feature Engineering
المؤلفون المشاركون
Derhab, Abdelouahid
Aldweesh, Arwa
Emam, Ahmed Z.
Khan, Farrukh Aslam
المصدر
Wireless Communications and Mobile Computing
العدد
المجلد 2020، العدد 2020 (31 ديسمبر/كانون الأول 2020)، ص ص. 1-16، 16ص.
الناشر
Hindawi Publishing Corporation
تاريخ النشر
2020-12-23
دولة النشر
مصر
عدد الصفحات
16
التخصصات الرئيسية
تكنولوجيا المعلومات وعلم الحاسوب
الملخص EN
In the era of the Internet of Things (IoT), connected objects produce an enormous amount of data traffic that feed big data analytics, which could be used in discovering unseen patterns and identifying anomalous traffic.
In this paper, we identify five key design principles that should be considered when developing a deep learning-based intrusion detection system (IDS) for the IoT.
Based on these principles, we design and implement Temporal Convolution Neural Network (TCNN), a deep learning framework for intrusion detection systems in IoT, which combines Convolution Neural Network (CNN) with causal convolution.
TCNN is combined with Synthetic Minority Oversampling Technique-Nominal Continuous (SMOTE-NC) to handle unbalanced dataset.
It is also combined with efficient feature engineering techniques, which consist of feature space reduction and feature transformation.
TCNN is evaluated on Bot-IoT dataset and compared with two common machine learning algorithms, i.e., Logistic Regression (LR) and Random Forest (RF), and two deep learning techniques, i.e., LSTM and CNN.
Experimental results show that TCNN achieves a good trade-off between effectiveness and efficiency.
It outperforms the state-of-the-art deep learning IDSs that are tested on Bot-IoT dataset and records an accuracy of 99.9986% for multiclass traffic detection, and shows a very close performance to CNN with respect to the training time.
نمط استشهاد جمعية علماء النفس الأمريكية (APA)
Derhab, Abdelouahid& Aldweesh, Arwa& Emam, Ahmed Z.& Khan, Farrukh Aslam. 2020. Intrusion Detection System for Internet of Things Based on Temporal Convolution Neural Network and Efficient Feature Engineering. Wireless Communications and Mobile Computing،Vol. 2020, no. 2020, pp.1-16.
https://search.emarefa.net/detail/BIM-1214494
نمط استشهاد الجمعية الأمريكية للغات الحديثة (MLA)
Derhab, Abdelouahid…[et al.]. Intrusion Detection System for Internet of Things Based on Temporal Convolution Neural Network and Efficient Feature Engineering. Wireless Communications and Mobile Computing No. 2020 (2020), pp.1-16.
https://search.emarefa.net/detail/BIM-1214494
نمط استشهاد الجمعية الطبية الأمريكية (AMA)
Derhab, Abdelouahid& Aldweesh, Arwa& Emam, Ahmed Z.& Khan, Farrukh Aslam. Intrusion Detection System for Internet of Things Based on Temporal Convolution Neural Network and Efficient Feature Engineering. Wireless Communications and Mobile Computing. 2020. Vol. 2020, no. 2020, pp.1-16.
https://search.emarefa.net/detail/BIM-1214494
نوع البيانات
مقالات
لغة النص
الإنجليزية
الملاحظات
Includes bibliographical references
رقم السجل
BIM-1214494
قاعدة معامل التأثير والاستشهادات المرجعية العربي "ارسيف Arcif"
أضخم قاعدة بيانات عربية للاستشهادات المرجعية للمجلات العلمية المحكمة الصادرة في العالم العربي

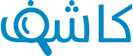
تقوم هذه الخدمة بالتحقق من التشابه أو الانتحال في الأبحاث والمقالات العلمية والأطروحات الجامعية والكتب والأبحاث باللغة العربية، وتحديد درجة التشابه أو أصالة الأعمال البحثية وحماية ملكيتها الفكرية. تعرف اكثر
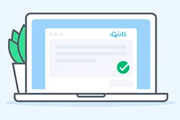