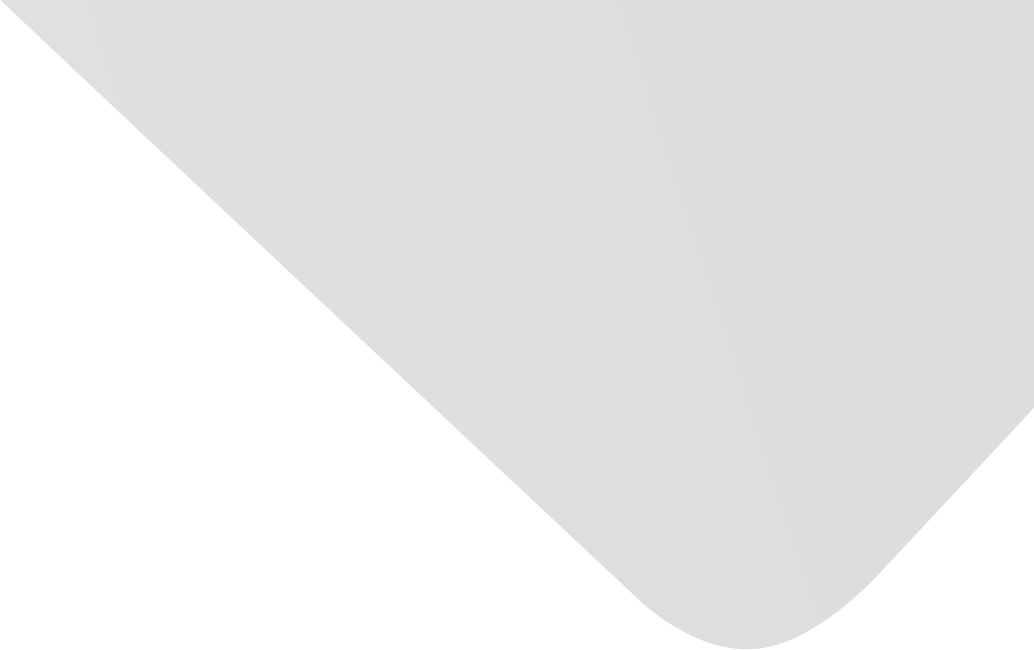
Intrusion Detection System for Internet of Things Based on Temporal Convolution Neural Network and Efficient Feature Engineering
Joint Authors
Derhab, Abdelouahid
Aldweesh, Arwa
Emam, Ahmed Z.
Khan, Farrukh Aslam
Source
Wireless Communications and Mobile Computing
Issue
Vol. 2020, Issue 2020 (31 Dec. 2020), pp.1-16, 16 p.
Publisher
Hindawi Publishing Corporation
Publication Date
2020-12-23
Country of Publication
Egypt
No. of Pages
16
Main Subjects
Information Technology and Computer Science
Abstract EN
In the era of the Internet of Things (IoT), connected objects produce an enormous amount of data traffic that feed big data analytics, which could be used in discovering unseen patterns and identifying anomalous traffic.
In this paper, we identify five key design principles that should be considered when developing a deep learning-based intrusion detection system (IDS) for the IoT.
Based on these principles, we design and implement Temporal Convolution Neural Network (TCNN), a deep learning framework for intrusion detection systems in IoT, which combines Convolution Neural Network (CNN) with causal convolution.
TCNN is combined with Synthetic Minority Oversampling Technique-Nominal Continuous (SMOTE-NC) to handle unbalanced dataset.
It is also combined with efficient feature engineering techniques, which consist of feature space reduction and feature transformation.
TCNN is evaluated on Bot-IoT dataset and compared with two common machine learning algorithms, i.e., Logistic Regression (LR) and Random Forest (RF), and two deep learning techniques, i.e., LSTM and CNN.
Experimental results show that TCNN achieves a good trade-off between effectiveness and efficiency.
It outperforms the state-of-the-art deep learning IDSs that are tested on Bot-IoT dataset and records an accuracy of 99.9986% for multiclass traffic detection, and shows a very close performance to CNN with respect to the training time.
American Psychological Association (APA)
Derhab, Abdelouahid& Aldweesh, Arwa& Emam, Ahmed Z.& Khan, Farrukh Aslam. 2020. Intrusion Detection System for Internet of Things Based on Temporal Convolution Neural Network and Efficient Feature Engineering. Wireless Communications and Mobile Computing،Vol. 2020, no. 2020, pp.1-16.
https://search.emarefa.net/detail/BIM-1214494
Modern Language Association (MLA)
Derhab, Abdelouahid…[et al.]. Intrusion Detection System for Internet of Things Based on Temporal Convolution Neural Network and Efficient Feature Engineering. Wireless Communications and Mobile Computing No. 2020 (2020), pp.1-16.
https://search.emarefa.net/detail/BIM-1214494
American Medical Association (AMA)
Derhab, Abdelouahid& Aldweesh, Arwa& Emam, Ahmed Z.& Khan, Farrukh Aslam. Intrusion Detection System for Internet of Things Based on Temporal Convolution Neural Network and Efficient Feature Engineering. Wireless Communications and Mobile Computing. 2020. Vol. 2020, no. 2020, pp.1-16.
https://search.emarefa.net/detail/BIM-1214494
Data Type
Journal Articles
Language
English
Notes
Includes bibliographical references
Record ID
BIM-1214494