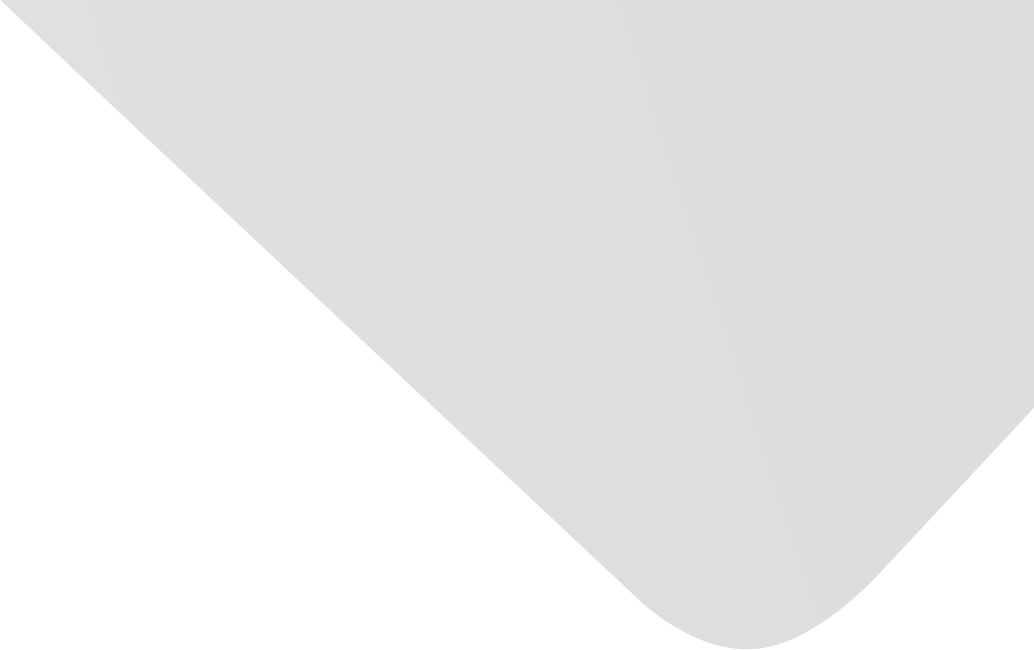
Intelligent sensor fault detection based on soft computing
العناوين الأخرى
كشف أخطاء الحساسات الذكية بالاستناد إلى نظم الحوسبة المرنة
المؤلفون المشاركون
Isa, Abbas Husayn
Majid, Ali Husayn
المصدر
العدد
المجلد 4، العدد 1 (30 يونيو/حزيران 2012)، ص ص. 113-123، 11ص.
الناشر
تاريخ النشر
2012-06-30
دولة النشر
العراق
عدد الصفحات
11
التخصصات الرئيسية
تكنولوجيا المعلومات وعلم الحاسوب
الموضوعات
الملخص AR
تم الكشف عن العطل في متحسس بالاستناد إلى خصائص الشبكة العصبية و نظام الاستدلال الضبابي العصبي التكيفي.
في هذا البحث تم استخدام الشبكة العصبية (تقنية لا تستند إلى النموذج الرياضي) و نظام الاستدلال الضبابي العصبي التكيفي ( ( ANFISللكشف عن العطل في متحسس درجة الحرارة .
TMP36 ثم بعد ذلك تم مقارنة قياس الخرج للمتحسس الحقيقي مع قراءات النموذج التي تم بناؤها و إذا ما تجاوز الفرق قيمة العتبة.
إن القيمة المتبقية من عملية المقارنة (الفرق) لها دور أساسي في مخططات كشف العيب (العطل) المستندة إلى نموذج.
في هذا البحث تم تطوير و تطبيق شبكة عصبية و نظام الاستدلال الضبابي العصبي التكيفي للتعامل مع الأنظمة اللاخطية بالاستناد إلى القيمة المتبقية لأغراض كشف العيب..إن الشبكتين تم اختبارهما على نموذج متحسس درجة الحرارة و مقارنة أداء كل من الشبكة العصية و نظام الاستدلال الضبابي العصبي التكيفي.
الملخص EN
Sensor fault detection is carried out based on the characteristics of the soft computing techniques; neural network and adaptive neural fuzzy inference system ANFIS.
In this paper, a neural network (non-model based technique) and ANFIS has been used for detection and isolation of temperature sensor fault TMP36.
The measured states are then compared with true estimated states and if their difference exceeds threshold value, the particular sensor measurement is ignored and replaced by the true estimated state.
Residual generation is an essential part of model-based fault detection schemes.
This paper develops and implements neural-network and ANFIS based system identification techniques for nonlinear systems with the specific goal of residual generation for fault detection purposes.
The two approaches are tested on a temperature sensor model.
Performance comparisons of the two neural network and ANFIS are presented.
نمط استشهاد جمعية علماء النفس الأمريكية (APA)
Isa, Abbas Husayn& Majid, Ali Husayn. 2012. Intelligent sensor fault detection based on soft computing. Kufa Journal of Engineering،Vol. 4, no. 1, pp.113-123.
https://search.emarefa.net/detail/BIM-329598
نمط استشهاد الجمعية الأمريكية للغات الحديثة (MLA)
Isa, Abbas Husayn& Majid, Ali Husayn. Intelligent sensor fault detection based on soft computing. Kufa Journal of Engineering Vol. 4, no. 1 (2012), pp.113-123.
https://search.emarefa.net/detail/BIM-329598
نمط استشهاد الجمعية الطبية الأمريكية (AMA)
Isa, Abbas Husayn& Majid, Ali Husayn. Intelligent sensor fault detection based on soft computing. Kufa Journal of Engineering. 2012. Vol. 4, no. 1, pp.113-123.
https://search.emarefa.net/detail/BIM-329598
نوع البيانات
مقالات
لغة النص
الإنجليزية
الملاحظات
Includes bibliographical references : p. 123
رقم السجل
BIM-329598
قاعدة معامل التأثير والاستشهادات المرجعية العربي "ارسيف Arcif"
أضخم قاعدة بيانات عربية للاستشهادات المرجعية للمجلات العلمية المحكمة الصادرة في العالم العربي

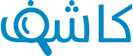
تقوم هذه الخدمة بالتحقق من التشابه أو الانتحال في الأبحاث والمقالات العلمية والأطروحات الجامعية والكتب والأبحاث باللغة العربية، وتحديد درجة التشابه أو أصالة الأعمال البحثية وحماية ملكيتها الفكرية. تعرف اكثر
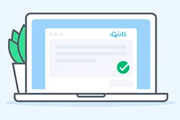