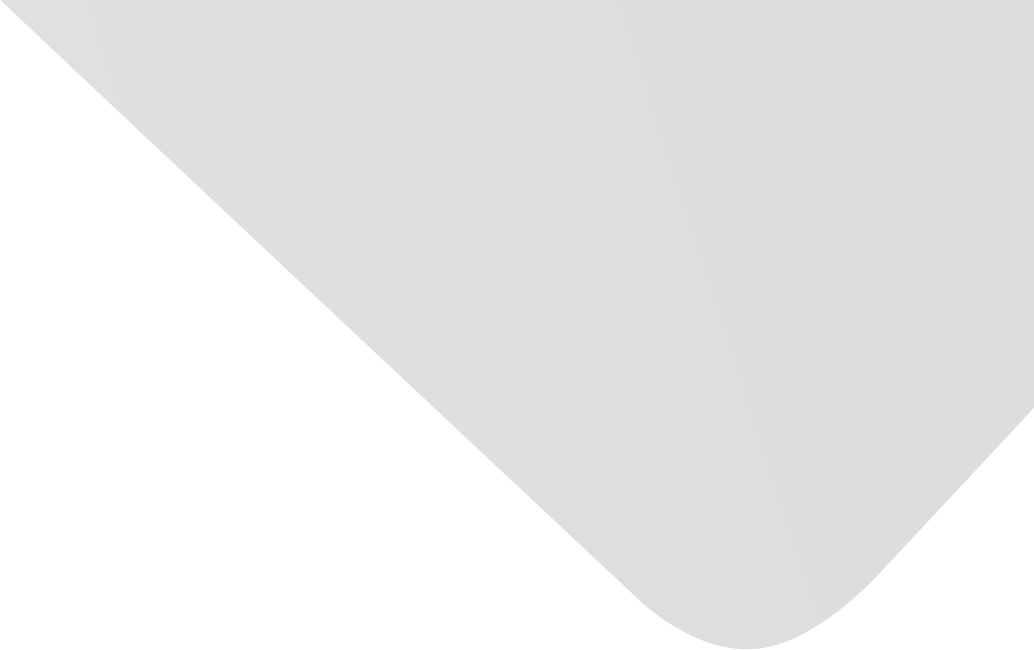
Intelligent sensor fault detection based on soft computing
Other Title(s)
كشف أخطاء الحساسات الذكية بالاستناد إلى نظم الحوسبة المرنة
Joint Authors
Isa, Abbas Husayn
Majid, Ali Husayn
Source
Issue
Vol. 4, Issue 1 (30 Jun. 2012), pp.113-123, 11 p.
Publisher
University of Kufa Faculty of Engineering
Publication Date
2012-06-30
Country of Publication
Iraq
No. of Pages
11
Main Subjects
Information Technology and Computer Science
Topics
Abstract AR
تم الكشف عن العطل في متحسس بالاستناد إلى خصائص الشبكة العصبية و نظام الاستدلال الضبابي العصبي التكيفي.
في هذا البحث تم استخدام الشبكة العصبية (تقنية لا تستند إلى النموذج الرياضي) و نظام الاستدلال الضبابي العصبي التكيفي ( ( ANFISللكشف عن العطل في متحسس درجة الحرارة .
TMP36 ثم بعد ذلك تم مقارنة قياس الخرج للمتحسس الحقيقي مع قراءات النموذج التي تم بناؤها و إذا ما تجاوز الفرق قيمة العتبة.
إن القيمة المتبقية من عملية المقارنة (الفرق) لها دور أساسي في مخططات كشف العيب (العطل) المستندة إلى نموذج.
في هذا البحث تم تطوير و تطبيق شبكة عصبية و نظام الاستدلال الضبابي العصبي التكيفي للتعامل مع الأنظمة اللاخطية بالاستناد إلى القيمة المتبقية لأغراض كشف العيب..إن الشبكتين تم اختبارهما على نموذج متحسس درجة الحرارة و مقارنة أداء كل من الشبكة العصية و نظام الاستدلال الضبابي العصبي التكيفي.
Abstract EN
Sensor fault detection is carried out based on the characteristics of the soft computing techniques; neural network and adaptive neural fuzzy inference system ANFIS.
In this paper, a neural network (non-model based technique) and ANFIS has been used for detection and isolation of temperature sensor fault TMP36.
The measured states are then compared with true estimated states and if their difference exceeds threshold value, the particular sensor measurement is ignored and replaced by the true estimated state.
Residual generation is an essential part of model-based fault detection schemes.
This paper develops and implements neural-network and ANFIS based system identification techniques for nonlinear systems with the specific goal of residual generation for fault detection purposes.
The two approaches are tested on a temperature sensor model.
Performance comparisons of the two neural network and ANFIS are presented.
American Psychological Association (APA)
Isa, Abbas Husayn& Majid, Ali Husayn. 2012. Intelligent sensor fault detection based on soft computing. Kufa Journal of Engineering،Vol. 4, no. 1, pp.113-123.
https://search.emarefa.net/detail/BIM-329598
Modern Language Association (MLA)
Isa, Abbas Husayn& Majid, Ali Husayn. Intelligent sensor fault detection based on soft computing. Kufa Journal of Engineering Vol. 4, no. 1 (2012), pp.113-123.
https://search.emarefa.net/detail/BIM-329598
American Medical Association (AMA)
Isa, Abbas Husayn& Majid, Ali Husayn. Intelligent sensor fault detection based on soft computing. Kufa Journal of Engineering. 2012. Vol. 4, no. 1, pp.113-123.
https://search.emarefa.net/detail/BIM-329598
Data Type
Journal Articles
Language
English
Notes
Includes bibliographical references : p. 123
Record ID
BIM-329598