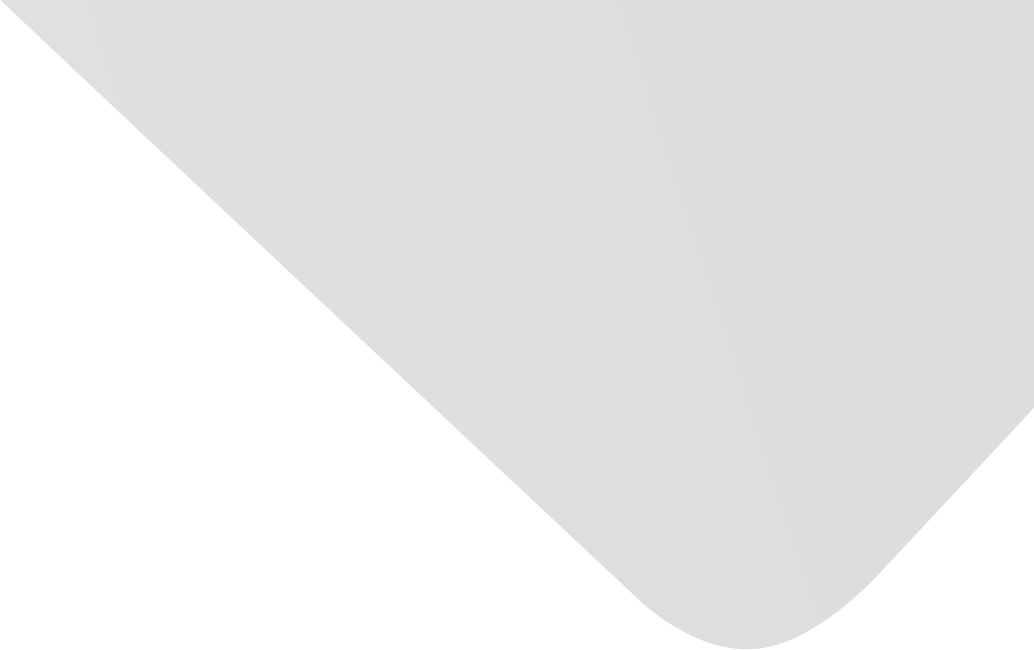
Combined First- and Second-Order Variational Model for Image Compressive Sensing
Joint Authors
Feng, Can
Xiao, Liang
Wei, Zhi-Hui
Source
Mathematical Problems in Engineering
Issue
Vol. 2013, Issue 2013 (31 Dec. 2013), pp.1-11, 11 p.
Publisher
Hindawi Publishing Corporation
Publication Date
2013-10-23
Country of Publication
Egypt
No. of Pages
11
Main Subjects
Abstract EN
A hybrid variational model combined first- and second-order total variation for image reconstruction from its finite number of noisy compressive samples is proposed in this paper.
Inspired by majorization-minimization scheme, we develop an efficient algorithm to seek the optimal solution of the proposed model by successively minimizing a sequence of quadratic surrogate penalties.
Both the nature and magnetic resonance (MR) images are used to compare its numerical performance with four state-of-the-art algorithms.
Experimental results demonstrate that the proposed algorithm obtained a significant improvement over related state-of-the-art algorithms in terms of the reconstruction relative error (RE) and peak signal to noise ratio (PSNR).
American Psychological Association (APA)
Feng, Can& Xiao, Liang& Wei, Zhi-Hui. 2013. Combined First- and Second-Order Variational Model for Image Compressive Sensing. Mathematical Problems in Engineering،Vol. 2013, no. 2013, pp.1-11.
https://search.emarefa.net/detail/BIM-1009487
Modern Language Association (MLA)
Feng, Can…[et al.]. Combined First- and Second-Order Variational Model for Image Compressive Sensing. Mathematical Problems in Engineering No. 2013 (2013), pp.1-11.
https://search.emarefa.net/detail/BIM-1009487
American Medical Association (AMA)
Feng, Can& Xiao, Liang& Wei, Zhi-Hui. Combined First- and Second-Order Variational Model for Image Compressive Sensing. Mathematical Problems in Engineering. 2013. Vol. 2013, no. 2013, pp.1-11.
https://search.emarefa.net/detail/BIM-1009487
Data Type
Journal Articles
Language
English
Notes
Includes bibliographical references
Record ID
BIM-1009487