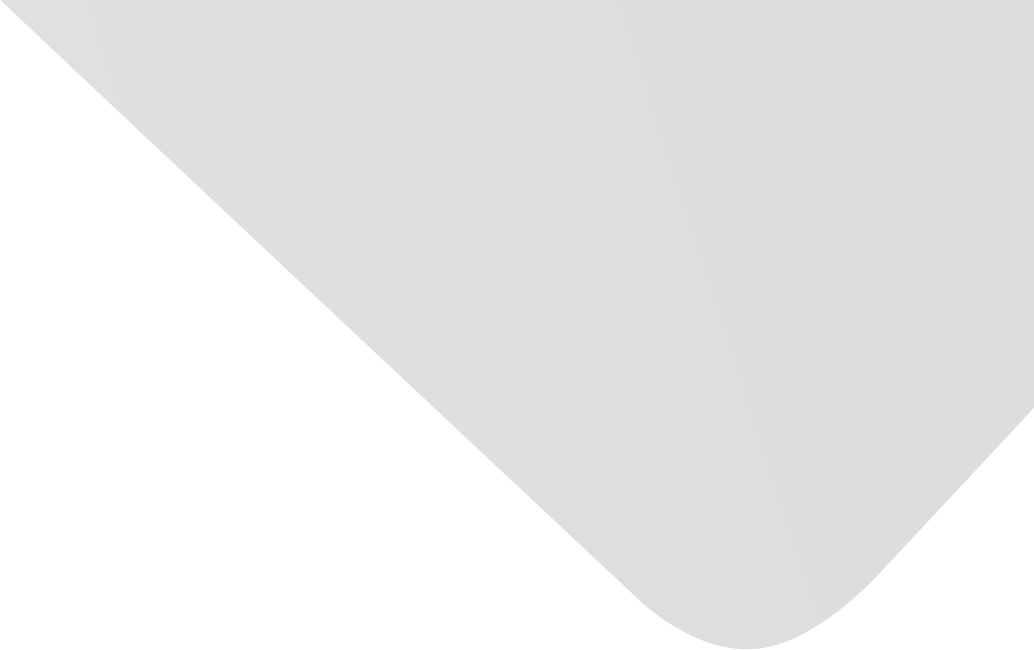
Protein Binding Site Prediction by Combining Hidden Markov Support Vector Machine and Profile-Based Propensities
Joint Authors
Wang, Xiaolong
Liu, Bin
Liu, Bingquan
Liu, Fule
Source
Issue
Vol. 2014, Issue 2014 (31 Dec. 2014), pp.1-6, 6 p.
Publisher
Hindawi Publishing Corporation
Publication Date
2014-07-13
Country of Publication
Egypt
No. of Pages
6
Main Subjects
Medicine
Information Technology and Computer Science
Abstract EN
Identification of protein binding sites is critical for studying the function of the proteins.
In this paper, we proposed a method for protein binding site prediction, which combined the order profile propensities and hidden Markov support vector machine (HM-SVM).
This method employed the sequential labeling technique to the field of protein binding site prediction.
The input features of HM-SVM include the profile-based propensities, the Position-Specific Score Matrix (PSSM), and Accessible Surface Area (ASA).
When tested on different data sets, the proposed method showed promising results, and outperformed some closely relative methods by more than 10% in terms of AUC.
American Psychological Association (APA)
Liu, Bin& Liu, Bingquan& Liu, Fule& Wang, Xiaolong. 2014. Protein Binding Site Prediction by Combining Hidden Markov Support Vector Machine and Profile-Based Propensities. The Scientific World Journal،Vol. 2014, no. 2014, pp.1-6.
https://search.emarefa.net/detail/BIM-1049716
Modern Language Association (MLA)
Liu, Bin…[et al.]. Protein Binding Site Prediction by Combining Hidden Markov Support Vector Machine and Profile-Based Propensities. The Scientific World Journal No. 2014 (2014), pp.1-6.
https://search.emarefa.net/detail/BIM-1049716
American Medical Association (AMA)
Liu, Bin& Liu, Bingquan& Liu, Fule& Wang, Xiaolong. Protein Binding Site Prediction by Combining Hidden Markov Support Vector Machine and Profile-Based Propensities. The Scientific World Journal. 2014. Vol. 2014, no. 2014, pp.1-6.
https://search.emarefa.net/detail/BIM-1049716
Data Type
Journal Articles
Language
English
Notes
Includes bibliographical references
Record ID
BIM-1049716