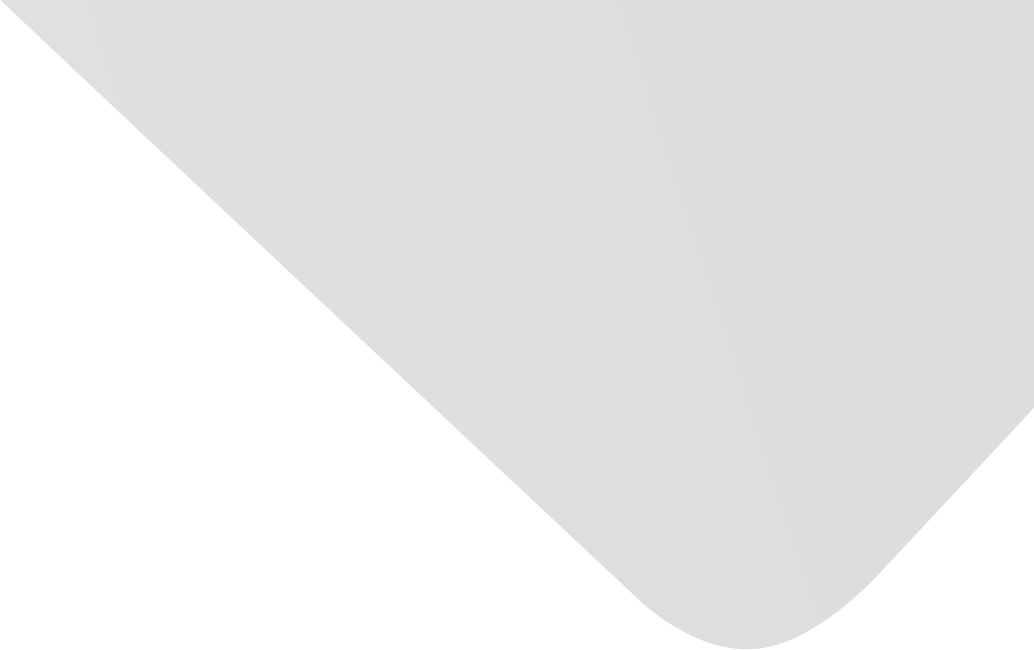
PSO-Based Support Vector Machine with Cuckoo Search Technique for Clinical Disease Diagnoses
Joint Authors
Source
Issue
Vol. 2014, Issue 2014 (31 Dec. 2014), pp.1-7, 7 p.
Publisher
Hindawi Publishing Corporation
Publication Date
2014-05-25
Country of Publication
Egypt
No. of Pages
7
Main Subjects
Medicine
Information Technology and Computer Science
Abstract EN
Disease diagnosis is conducted with a machine learning method.
We have proposed a novel machine learning method that hybridizes support vector machine (SVM), particle swarm optimization (PSO), and cuckoo search (CS).
The new method consists of two stages: firstly, a CS based approach for parameter optimization of SVM is developed to find the better initial parameters of kernel function, and then PSO is applied to continue SVM training and find the best parameters of SVM.
Experimental results indicate that the proposed CS-PSO-SVM model achieves better classification accuracy and F-measure than PSO-SVM and GA-SVM.
Therefore, we can conclude that our proposed method is very efficient compared to the previously reported algorithms.
American Psychological Association (APA)
Liu, Xiaoyong& Fu, Hui. 2014. PSO-Based Support Vector Machine with Cuckoo Search Technique for Clinical Disease Diagnoses. The Scientific World Journal،Vol. 2014, no. 2014, pp.1-7.
https://search.emarefa.net/detail/BIM-1050086
Modern Language Association (MLA)
Liu, Xiaoyong& Fu, Hui. PSO-Based Support Vector Machine with Cuckoo Search Technique for Clinical Disease Diagnoses. The Scientific World Journal No. 2014 (2014), pp.1-7.
https://search.emarefa.net/detail/BIM-1050086
American Medical Association (AMA)
Liu, Xiaoyong& Fu, Hui. PSO-Based Support Vector Machine with Cuckoo Search Technique for Clinical Disease Diagnoses. The Scientific World Journal. 2014. Vol. 2014, no. 2014, pp.1-7.
https://search.emarefa.net/detail/BIM-1050086
Data Type
Journal Articles
Language
English
Notes
Includes bibliographical references
Record ID
BIM-1050086