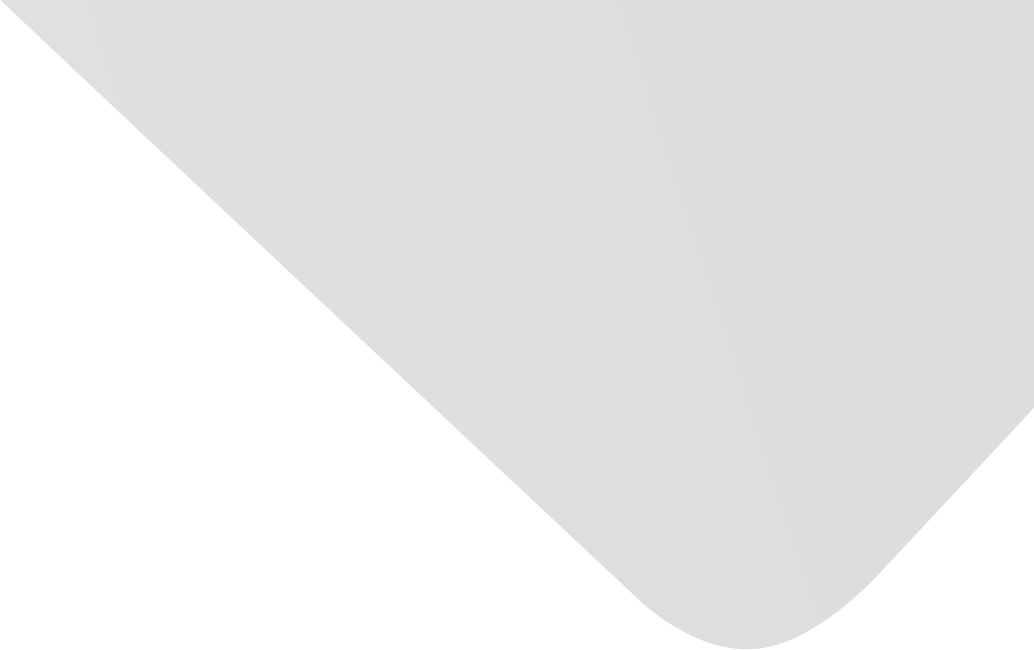
A Shortest Dependency Path Based Convolutional Neural Network for Protein-Protein Relation Extraction
Joint Authors
Source
Issue
Vol. 2016, Issue 2016 (31 Dec. 2016), pp.1-9, 9 p.
Publisher
Hindawi Publishing Corporation
Publication Date
2016-07-14
Country of Publication
Egypt
No. of Pages
9
Main Subjects
Abstract EN
The state-of-the-art methods for protein-protein interaction (PPI) extraction are primarily based on kernel methods, and their performances strongly depend on the handcraft features.
In this paper, we tackle PPI extraction by using convolutional neural networks (CNN) and propose a shortest dependency path based CNN (sdpCNN) model.
The proposed method ( 1 ) only takes the sdp and word embedding as input and ( 2 ) could avoid bias from feature selection by using CNN.
We performed experiments on standard Aimed and BioInfer datasets, and the experimental results demonstrated that our approach outperformed state-of-the-art kernel based methods.
In particular, by tracking the sdpCNN model, we find that sdpCNN could extract key features automatically and it is verified that pretrained word embedding is crucial in PPI task.
American Psychological Association (APA)
Hua, Lei& Quan, Chanqin. 2016. A Shortest Dependency Path Based Convolutional Neural Network for Protein-Protein Relation Extraction. BioMed Research International،Vol. 2016, no. 2016, pp.1-9.
https://search.emarefa.net/detail/BIM-1099067
Modern Language Association (MLA)
Hua, Lei& Quan, Chanqin. A Shortest Dependency Path Based Convolutional Neural Network for Protein-Protein Relation Extraction. BioMed Research International No. 2016 (2016), pp.1-9.
https://search.emarefa.net/detail/BIM-1099067
American Medical Association (AMA)
Hua, Lei& Quan, Chanqin. A Shortest Dependency Path Based Convolutional Neural Network for Protein-Protein Relation Extraction. BioMed Research International. 2016. Vol. 2016, no. 2016, pp.1-9.
https://search.emarefa.net/detail/BIM-1099067
Data Type
Journal Articles
Language
English
Notes
Includes bibliographical references
Record ID
BIM-1099067