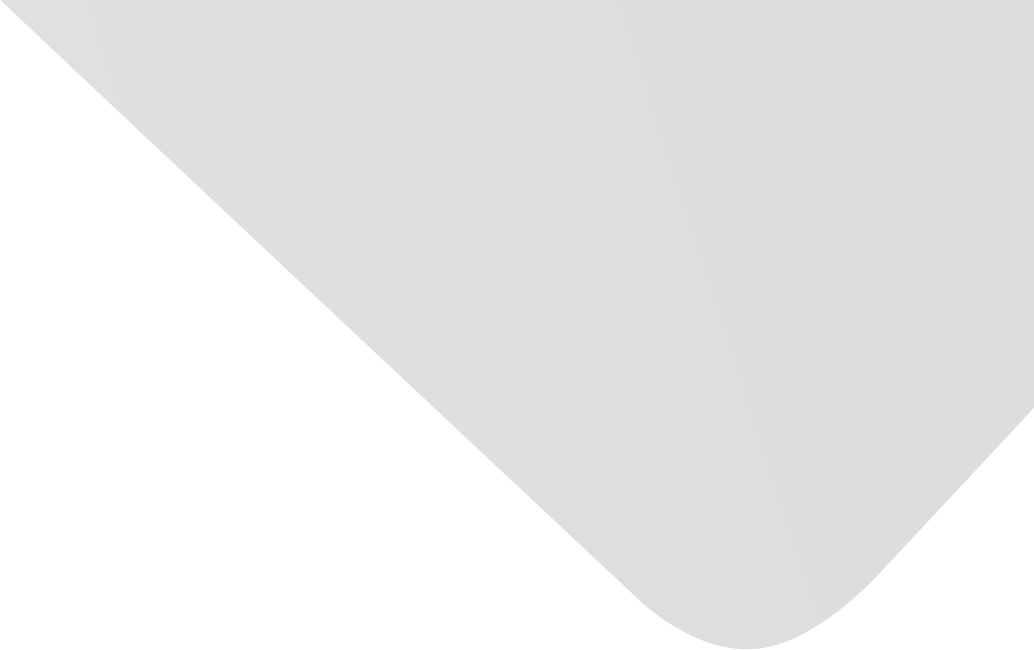
Weighted Feature Gaussian Kernel SVM for Emotion Recognition
Joint Authors
Source
Computational Intelligence and Neuroscience
Issue
Vol. 2016, Issue 2016 (31 Dec. 2015), pp.1-7, 7 p.
Publisher
Hindawi Publishing Corporation
Publication Date
2016-10-11
Country of Publication
Egypt
No. of Pages
7
Main Subjects
Abstract EN
Emotion recognition with weighted feature based on facial expression is a challenging research topic and has attracted great attention in the past few years.
This paper presents a novel method, utilizing subregion recognition rate to weight kernel function.
First, we divide the facial expression image into some uniform subregions and calculate corresponding recognition rate and weight.
Then, we get a weighted feature Gaussian kernel function and construct a classifier based on Support Vector Machine (SVM).
At last, the experimental results suggest that the approach based on weighted feature Gaussian kernel function has good performance on the correct rate in emotion recognition.
The experiments on the extended Cohn-Kanade (CK+) dataset show that our method has achieved encouraging recognition results compared to the state-of-the-art methods.
American Psychological Association (APA)
Wei, Wei& Jia, Qingxuan. 2016. Weighted Feature Gaussian Kernel SVM for Emotion Recognition. Computational Intelligence and Neuroscience،Vol. 2016, no. 2016, pp.1-7.
https://search.emarefa.net/detail/BIM-1099759
Modern Language Association (MLA)
Wei, Wei& Jia, Qingxuan. Weighted Feature Gaussian Kernel SVM for Emotion Recognition. Computational Intelligence and Neuroscience Vol. 2016, no. 2016 (2015), pp.1-7.
https://search.emarefa.net/detail/BIM-1099759
American Medical Association (AMA)
Wei, Wei& Jia, Qingxuan. Weighted Feature Gaussian Kernel SVM for Emotion Recognition. Computational Intelligence and Neuroscience. 2016. Vol. 2016, no. 2016, pp.1-7.
https://search.emarefa.net/detail/BIM-1099759
Data Type
Journal Articles
Language
English
Notes
Includes bibliographical references
Record ID
BIM-1099759