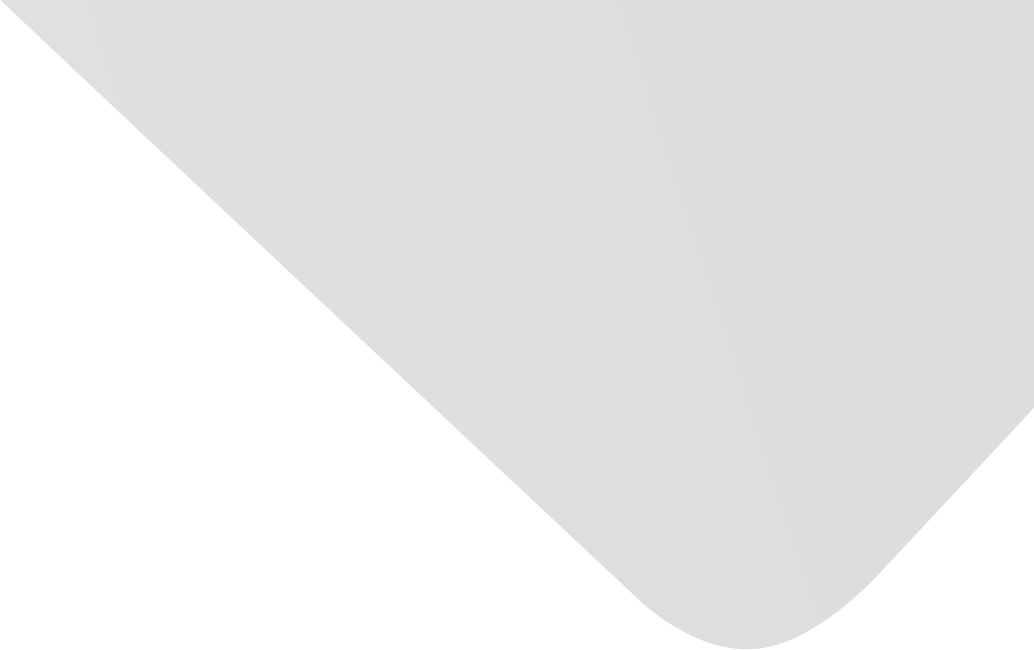
Prediction of G Protein-Coupled Receptors with SVM-Prot Features and Random Forest
Joint Authors
Source
Issue
Vol. 2016, Issue 2016 (31 Dec. 2016), pp.1-10, 10 p.
Publisher
Hindawi Publishing Corporation
Publication Date
2016-07-27
Country of Publication
Egypt
No. of Pages
10
Main Subjects
Abstract EN
G protein-coupled receptors (GPCRs) are the largest receptor superfamily.
In this paper, we try to employ physical-chemical properties, which come from SVM-Prot, to represent GPCR.
Random Forest was utilized as classifier for distinguishing them from other protein sequences.
MEME suite was used to detect the most significant 10 conserved motifs of human GPCRs.
In the testing datasets, the average accuracy was 91.61%, and the average AUC was 0.9282.
MEME discovery analysis showed that many motifs aggregated in the seven hydrophobic helices transmembrane regions adapt to the characteristic of GPCRs.
All of the above indicate that our machine-learning method can successfully distinguish GPCRs from non-GPCRs.
American Psychological Association (APA)
Liao, Zhijun& Ju, Ying& Zou, Quan. 2016. Prediction of G Protein-Coupled Receptors with SVM-Prot Features and Random Forest. Scientifica،Vol. 2016, no. 2016, pp.1-10.
https://search.emarefa.net/detail/BIM-1117911
Modern Language Association (MLA)
Liao, Zhijun…[et al.]. Prediction of G Protein-Coupled Receptors with SVM-Prot Features and Random Forest. Scientifica No. 2016 (2016), pp.1-10.
https://search.emarefa.net/detail/BIM-1117911
American Medical Association (AMA)
Liao, Zhijun& Ju, Ying& Zou, Quan. Prediction of G Protein-Coupled Receptors with SVM-Prot Features and Random Forest. Scientifica. 2016. Vol. 2016, no. 2016, pp.1-10.
https://search.emarefa.net/detail/BIM-1117911
Data Type
Journal Articles
Language
English
Notes
Includes bibliographical references
Record ID
BIM-1117911