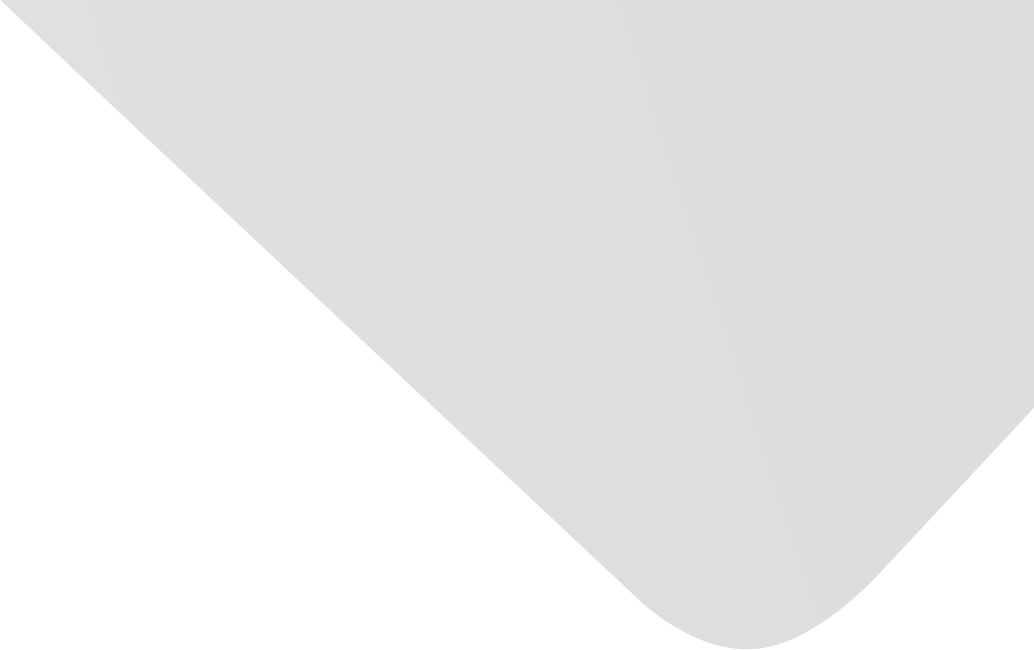
Background Information Self-Learning Based Hyperspectral Target Detection
Joint Authors
Tian, Yufei
Yang, Jihai
Li, Shijun
Xu, Wenning
Source
Issue
Vol. 2018, Issue 2018 (31 Dec. 2018), pp.1-7, 7 p.
Publisher
Hindawi Publishing Corporation
Publication Date
2018-07-03
Country of Publication
Egypt
No. of Pages
7
Main Subjects
Abstract EN
Hyperspectral imaging has been proved as an effective way to explore the useful information behind the land objects.
And it can also be adopted for biologic information extraction, by which the origin information can be acquired from the image repeatedly without contamination.
In this paper we proposed a target detection method based on background self-learning to extract the biologic information from the hyperspectral images.
The conventional unstructured target detectors are very difficult to estimate the background statistics accurately in either a global or local way.
Considering the spatial spectral information, its performance can be further improved by avoiding the above problem.
It is especially designed to extract fingerprint and tumor region from hyperspectral biologic images.
The experimental results show the validity and the superiority of our method on detecting the biologic information from hyperspectral images.
American Psychological Association (APA)
Tian, Yufei& Yang, Jihai& Li, Shijun& Xu, Wenning. 2018. Background Information Self-Learning Based Hyperspectral Target Detection. Complexity،Vol. 2018, no. 2018, pp.1-7.
https://search.emarefa.net/detail/BIM-1133645
Modern Language Association (MLA)
Tian, Yufei…[et al.]. Background Information Self-Learning Based Hyperspectral Target Detection. Complexity No. 2018 (2018), pp.1-7.
https://search.emarefa.net/detail/BIM-1133645
American Medical Association (AMA)
Tian, Yufei& Yang, Jihai& Li, Shijun& Xu, Wenning. Background Information Self-Learning Based Hyperspectral Target Detection. Complexity. 2018. Vol. 2018, no. 2018, pp.1-7.
https://search.emarefa.net/detail/BIM-1133645
Data Type
Journal Articles
Language
English
Notes
Includes bibliographical references
Record ID
BIM-1133645