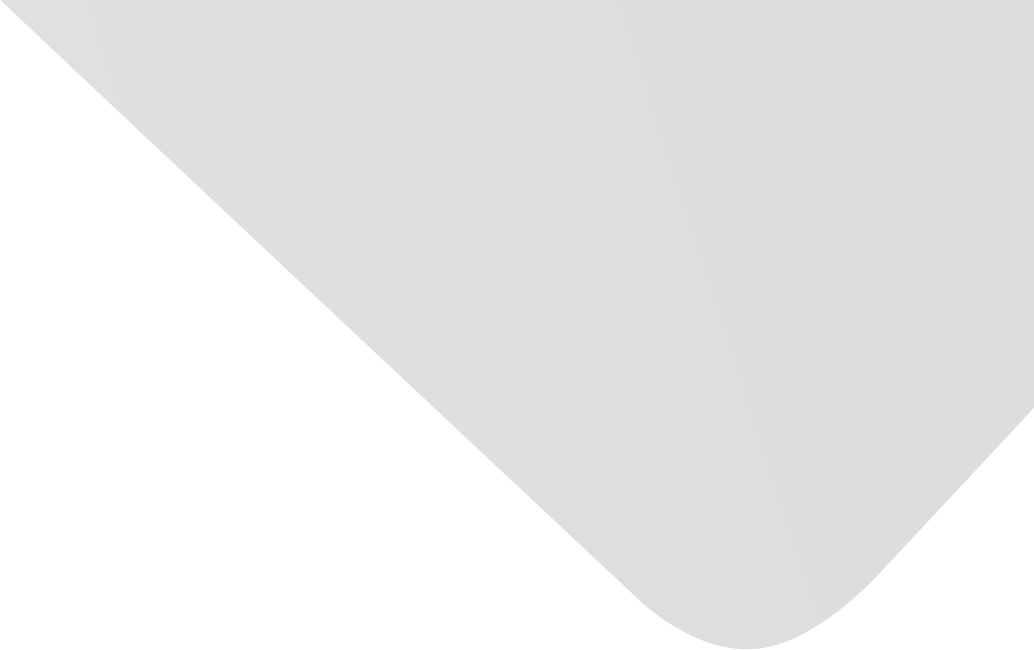
Change Detection of Remote Sensing Images Based on Attention Mechanism
Joint Authors
Li, Peng
Zhang, Dezheng
Lv, Peng
Chen, Long
Source
Computational Intelligence and Neuroscience
Issue
Vol. 2020, Issue 2020 (31 Dec. 2020), pp.1-11, 11 p.
Publisher
Hindawi Publishing Corporation
Publication Date
2020-08-25
Country of Publication
Egypt
No. of Pages
11
Main Subjects
Abstract EN
In recent years, image processing methods based on convolutional neural networks (CNNs) have achieved very good results.
At the same time, many branch techniques have been proposed to improve accuracy.
Aiming at the change detection task of remote sensing images, we propose a new network based on U-Net in this paper.
The attention mechanism is cleverly applied in the change detection task, and the data-dependent upsampling (DUpsampling) method is used at the same time, so that the network shows improvement in accuracy, and the calculation amount is greatly reduced.
The experimental results show that, in the two-phase images of Yinchuan City, the proposed network has a better antinoise ability and can avoid false detection to a certain extent.
American Psychological Association (APA)
Chen, Long& Zhang, Dezheng& Li, Peng& Lv, Peng. 2020. Change Detection of Remote Sensing Images Based on Attention Mechanism. Computational Intelligence and Neuroscience،Vol. 2020, no. 2020, pp.1-11.
https://search.emarefa.net/detail/BIM-1138782
Modern Language Association (MLA)
Chen, Long…[et al.]. Change Detection of Remote Sensing Images Based on Attention Mechanism. Computational Intelligence and Neuroscience No. 2020 (2020), pp.1-11.
https://search.emarefa.net/detail/BIM-1138782
American Medical Association (AMA)
Chen, Long& Zhang, Dezheng& Li, Peng& Lv, Peng. Change Detection of Remote Sensing Images Based on Attention Mechanism. Computational Intelligence and Neuroscience. 2020. Vol. 2020, no. 2020, pp.1-11.
https://search.emarefa.net/detail/BIM-1138782
Data Type
Journal Articles
Language
English
Notes
Includes bibliographical references
Record ID
BIM-1138782