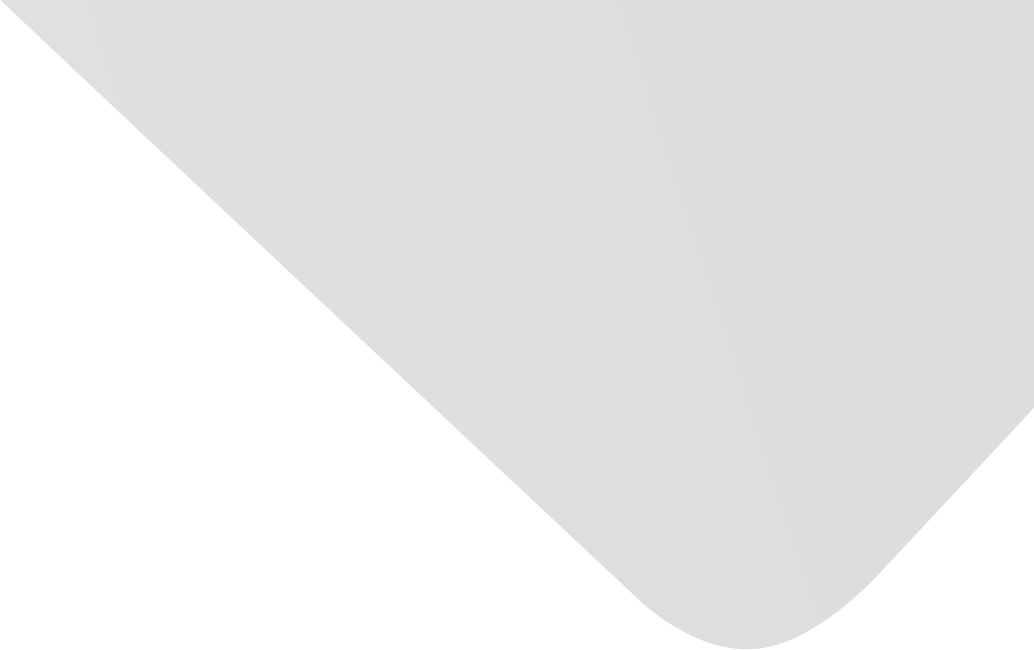
EEG-Based Epilepsy Recognition via Multiple Kernel Learning
Joint Authors
Yao, Yufeng
Cui, Zhiming
Ding, Yan
Zhong, Shan
Source
Computational and Mathematical Methods in Medicine
Issue
Vol. 2020, Issue 2020 (31 Dec. 2020), pp.1-9, 9 p.
Publisher
Hindawi Publishing Corporation
Publication Date
2020-09-29
Country of Publication
Egypt
No. of Pages
9
Main Subjects
Abstract EN
In the field of brain-computer interfaces, it is very common to use EEG signals for disease diagnosis.
In this study, a style regularized least squares support vector machine based on multikernel learning is proposed and applied to the recognition of epilepsy abnormal signals.
The algorithm uses the style conversion matrix to represent the style information contained in the sample, regularizes it in the objective function, optimizes the objective function through the commonly used alternative optimization method, and simultaneously updates the style conversion matrix and classifier during the iteration process parameter.
In order to use the learned style information in the prediction process, two new rules are added to the traditional prediction method, and the style conversion matrix is used to standardize the sample style before classification.
American Psychological Association (APA)
Yao, Yufeng& Ding, Yan& Zhong, Shan& Cui, Zhiming. 2020. EEG-Based Epilepsy Recognition via Multiple Kernel Learning. Computational and Mathematical Methods in Medicine،Vol. 2020, no. 2020, pp.1-9.
https://search.emarefa.net/detail/BIM-1139591
Modern Language Association (MLA)
Yao, Yufeng…[et al.]. EEG-Based Epilepsy Recognition via Multiple Kernel Learning. Computational and Mathematical Methods in Medicine No. 2020 (2020), pp.1-9.
https://search.emarefa.net/detail/BIM-1139591
American Medical Association (AMA)
Yao, Yufeng& Ding, Yan& Zhong, Shan& Cui, Zhiming. EEG-Based Epilepsy Recognition via Multiple Kernel Learning. Computational and Mathematical Methods in Medicine. 2020. Vol. 2020, no. 2020, pp.1-9.
https://search.emarefa.net/detail/BIM-1139591
Data Type
Journal Articles
Language
English
Notes
Includes bibliographical references
Record ID
BIM-1139591