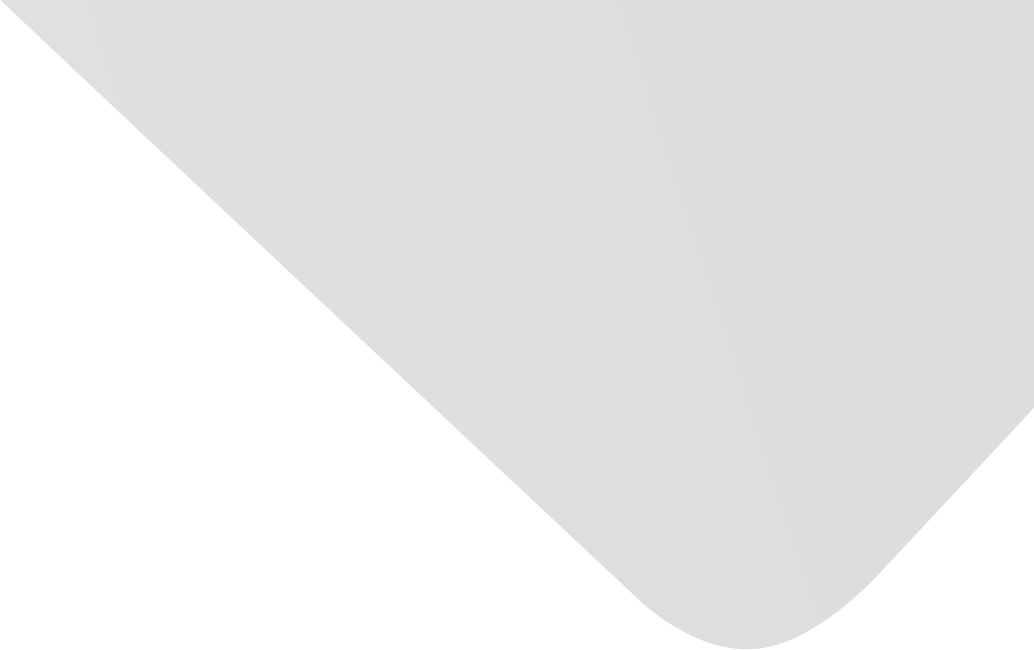
Block-Constraint Laplacian-Regularized Low-Rank Representation and Its Application for Cancer Sample Clustering Based on Integrated TCGA Data
Joint Authors
Zheng, Chun-Hou
Liu, Jin-Xing
Wang, Juan
Dai, Ling-Yun
Lu, Cong-Hai
Kong, Xiang-Zhen
Source
Issue
Vol. 2020, Issue 2020 (31 Dec. 2020), pp.1-13, 13 p.
Publisher
Hindawi Publishing Corporation
Publication Date
2020-01-27
Country of Publication
Egypt
No. of Pages
13
Main Subjects
Abstract EN
Low-Rank Representation (LRR) is a powerful subspace clustering method because of its successful learning of low-dimensional subspace of data.
With the breakthrough of “OMics” technology, many LRR-based methods have been proposed and used to cancer clustering based on gene expression data.
Moreover, studies have shown that besides gene expression data, some other genomic data in TCGA also contain important information for cancer research.
Therefore, these genomic data can be integrated as a comprehensive feature source for cancer clustering.
How to establish an effective clustering model for comprehensive analysis of integrated TCGA data has become a key issue.
In this paper, we develop the traditional LRR method and propose a novel method named Block-constraint Laplacian-Regularized Low-Rank Representation (BLLRR) to model multigenome data for cancer sample clustering.
The proposed method is dedicated to extracting more abundant subspace structure information from multiple genomic data to improve the accuracy of cancer sample clustering.
Considering the heterogeneity of different genome data, we introduce the block-constraint idea into our method.
In BLLRR decomposition, we treat each genome data as a data block and impose different constraints on different data blocks.
In addition, graph Laplacian is also introduced into our method to better learn the topological structure of data by preserving the local geometric information.
The experiments demonstrate that the BLLRR method can effectively analyze integrated TCGA data and extract more subspace structure information from multigenome data.
It is a reliable and efficient clustering algorithm for cancer sample clustering.
American Psychological Association (APA)
Wang, Juan& Liu, Jin-Xing& Zheng, Chun-Hou& Lu, Cong-Hai& Dai, Ling-Yun& Kong, Xiang-Zhen. 2020. Block-Constraint Laplacian-Regularized Low-Rank Representation and Its Application for Cancer Sample Clustering Based on Integrated TCGA Data. Complexity،Vol. 2020, no. 2020, pp.1-13.
https://search.emarefa.net/detail/BIM-1142114
Modern Language Association (MLA)
Wang, Juan…[et al.]. Block-Constraint Laplacian-Regularized Low-Rank Representation and Its Application for Cancer Sample Clustering Based on Integrated TCGA Data. Complexity No. 2020 (2020), pp.1-13.
https://search.emarefa.net/detail/BIM-1142114
American Medical Association (AMA)
Wang, Juan& Liu, Jin-Xing& Zheng, Chun-Hou& Lu, Cong-Hai& Dai, Ling-Yun& Kong, Xiang-Zhen. Block-Constraint Laplacian-Regularized Low-Rank Representation and Its Application for Cancer Sample Clustering Based on Integrated TCGA Data. Complexity. 2020. Vol. 2020, no. 2020, pp.1-13.
https://search.emarefa.net/detail/BIM-1142114
Data Type
Journal Articles
Language
English
Notes
Includes bibliographical references
Record ID
BIM-1142114