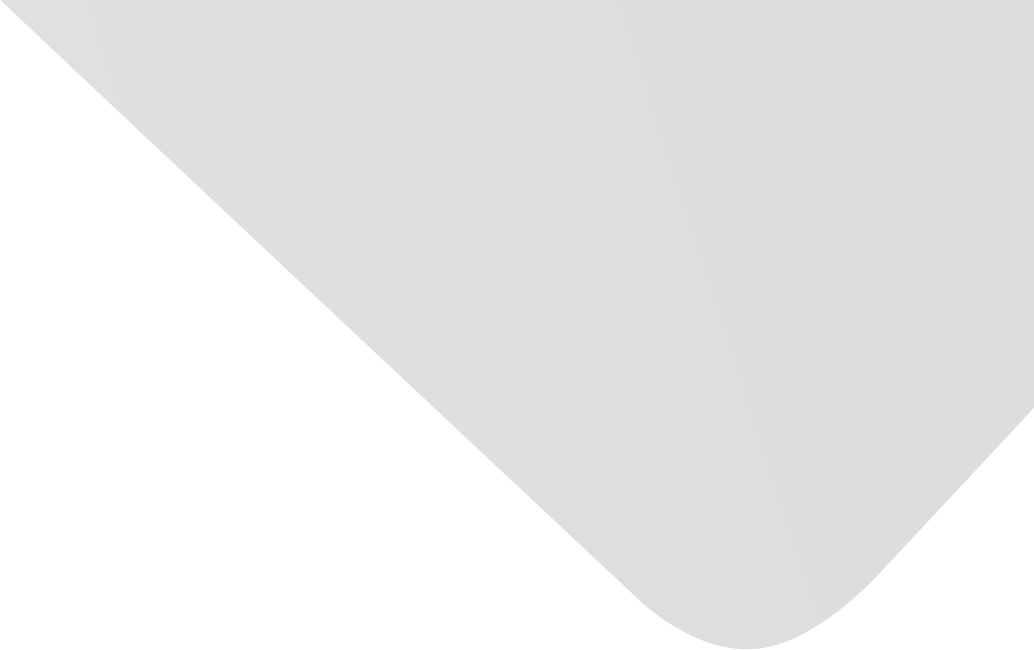
Social Network Community Detection Using Agglomerative Spectral Clustering
Joint Authors
Narantsatsralt, Ulzii-Utas
Kang, Sanggil
Source
Issue
Vol. 2017, Issue 2017 (31 Dec. 2017), pp.1-10, 10 p.
Publisher
Hindawi Publishing Corporation
Publication Date
2017-11-07
Country of Publication
Egypt
No. of Pages
10
Main Subjects
Abstract EN
Community detection has become an increasingly popular tool for analyzing and researching complex networks.
Many methods have been proposed for accurate community detection, and one of them is spectral clustering.
Most spectral clustering algorithms have been implemented on artificial networks, and accuracy of the community detection is still unsatisfactory.
Therefore, this paper proposes an agglomerative spectral clustering method with conductance and edge weights.
In this method, the most similar nodes are agglomerated based on eigenvector space and edge weights.
In addition, the conductance is used to identify densely connected clusters while agglomerating.
The proposed method shows improved performance in related works and proves to be efficient for real life complex networks from experiments.
American Psychological Association (APA)
Narantsatsralt, Ulzii-Utas& Kang, Sanggil. 2017. Social Network Community Detection Using Agglomerative Spectral Clustering. Complexity،Vol. 2017, no. 2017, pp.1-10.
https://search.emarefa.net/detail/BIM-1142752
Modern Language Association (MLA)
Narantsatsralt, Ulzii-Utas& Kang, Sanggil. Social Network Community Detection Using Agglomerative Spectral Clustering. Complexity No. 2017 (2017), pp.1-10.
https://search.emarefa.net/detail/BIM-1142752
American Medical Association (AMA)
Narantsatsralt, Ulzii-Utas& Kang, Sanggil. Social Network Community Detection Using Agglomerative Spectral Clustering. Complexity. 2017. Vol. 2017, no. 2017, pp.1-10.
https://search.emarefa.net/detail/BIM-1142752
Data Type
Journal Articles
Language
English
Notes
Includes bibliographical references
Record ID
BIM-1142752