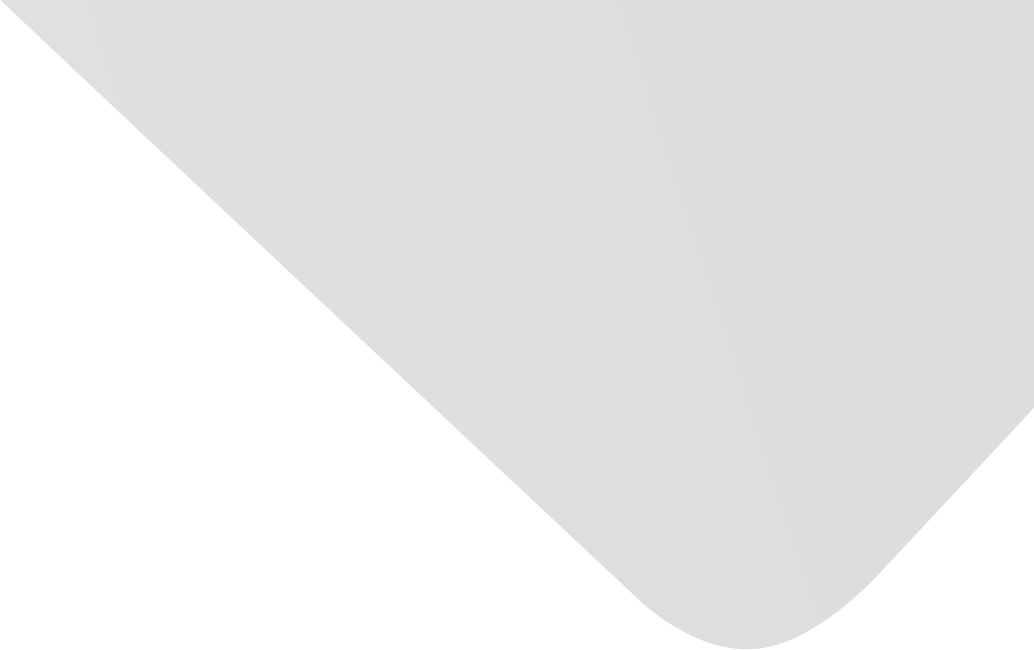
Multiple Morphological Constraints-Based Complex Gland Segmentation in Colorectal Cancer Pathology Image Analysis
Joint Authors
Hua, Liang
Zhou, H.
Zhang, Kun
Fu, JunHong
Zhang, Peijian
Shao, Yeqin
Xu, Sheng
Wang, Jing
Chen, Li
Source
Issue
Vol. 2020, Issue 2020 (31 Dec. 2020), pp.1-16, 16 p.
Publisher
Hindawi Publishing Corporation
Publication Date
2020-07-28
Country of Publication
Egypt
No. of Pages
16
Main Subjects
Abstract EN
Histological assessment of glands is one of the major concerns in colon cancer grading.
Considering that poorly differentiated colorectal glands cannot be accurately segmented, we propose an approach for segmentation of glands in colon cancer images, based on the characteristics of lumens and rough gland boundaries.
First, we use a U-net for stain separation to obtain H-stain, E-stain, and background stain intensity maps.
Subsequently, epithelial nucleus is identified on the histopathology images, and the lumen segmentation is performed on the background intensity map.
Then, we use the axis of least inertia-based similar triangles as the spatial characteristics of lumens and epithelial nucleus, and a triangle membership is used to select glandular contour candidates from epithelial nucleus.
By connecting lumens and epithelial nucleus, more accurate gland segmentation is performed based on the rough gland boundary.
The proposed stain separation approach is unsupervised, and the stain separation makes the category information contained in the H&E image easy to identify and deal with the uneven stain intensity and the inconspicuous stain difference.
In this project, we use deep learning to achieve stain separation by predicting the stain coefficient.
Under the deep learning framework, we design a stain coefficient interval model to improve the stain generalization performance.
Another innovation is that we propose the combination of the internal lumen contour of adenoma and the outer contour of epithelial cells to obtain a precise gland contour.
We compare the performance of the proposed algorithm against that of several state-of-the-art technologies on publicly available datasets.
The results show that the segmentation approach combining the characteristics of lumens and rough gland boundary has better segmentation accuracy.
American Psychological Association (APA)
Zhang, Kun& Fu, JunHong& Hua, Liang& Zhang, Peijian& Shao, Yeqin& Xu, Sheng…[et al.]. 2020. Multiple Morphological Constraints-Based Complex Gland Segmentation in Colorectal Cancer Pathology Image Analysis. Complexity،Vol. 2020, no. 2020, pp.1-16.
https://search.emarefa.net/detail/BIM-1142765
Modern Language Association (MLA)
Zhang, Kun…[et al.]. Multiple Morphological Constraints-Based Complex Gland Segmentation in Colorectal Cancer Pathology Image Analysis. Complexity No. 2020 (2020), pp.1-16.
https://search.emarefa.net/detail/BIM-1142765
American Medical Association (AMA)
Zhang, Kun& Fu, JunHong& Hua, Liang& Zhang, Peijian& Shao, Yeqin& Xu, Sheng…[et al.]. Multiple Morphological Constraints-Based Complex Gland Segmentation in Colorectal Cancer Pathology Image Analysis. Complexity. 2020. Vol. 2020, no. 2020, pp.1-16.
https://search.emarefa.net/detail/BIM-1142765
Data Type
Journal Articles
Language
English
Notes
Includes bibliographical references
Record ID
BIM-1142765