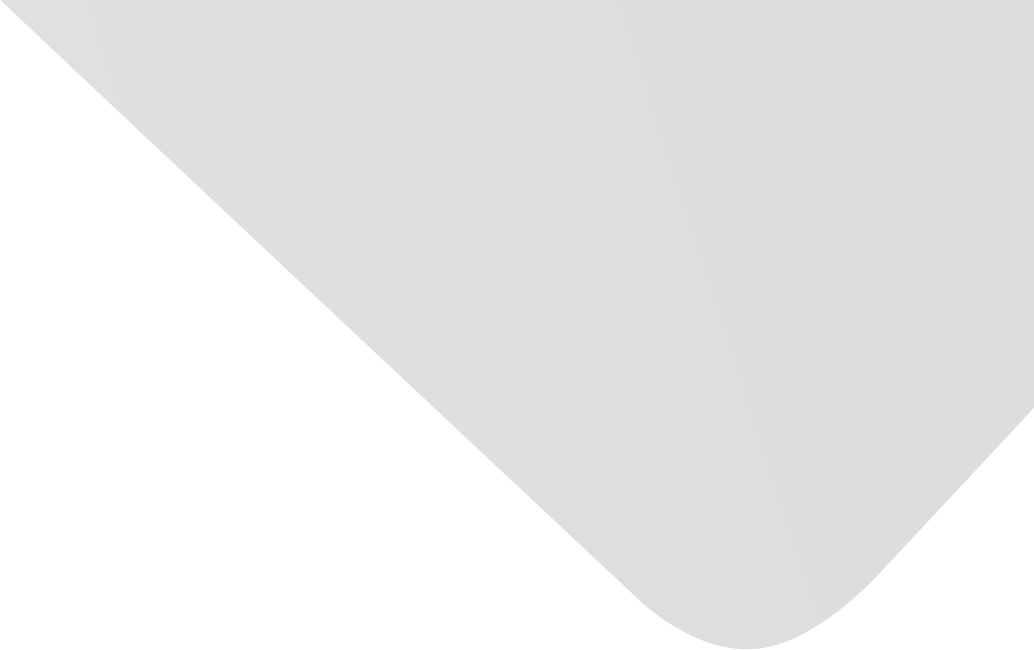
M&A Short-Term Performance Based on Elman Neural Network Model: Evidence from 2006 to 2019 in China
Joint Authors
Xiao, Ming
Yang, Xionghui
Li, Ge
Source
Issue
Vol. 2020, Issue 2020 (31 Dec. 2020), pp.1-15, 15 p.
Publisher
Hindawi Publishing Corporation
Publication Date
2020-12-12
Country of Publication
Egypt
No. of Pages
15
Main Subjects
Abstract EN
Based on the event study method, this paper conducts the analysis on the short-term performance of 1302 major mergers and acquisitions (M&A) in China from 2006 to 2019 and takes the cumulative abnormal return (CAR) as the measurement index.
After comparing the five abnormal return (AR) calculation models, it is found that the commonly used market model method and the market adjustment method have statistical defects while the Elman feedback neural network model is capable of good nonlinear prediction ability.
The study shows that M&A can create considerable short-term performance for Chinese listed company shareholders.
The CAR in window period reached 14.45% with a downward trend, which is the win-win result achieved through the cooperation between multiple parties and individuals driven by their respective rights and interests in the current macro-microeconomic environment in China.
American Psychological Association (APA)
Xiao, Ming& Yang, Xionghui& Li, Ge. 2020. M&A Short-Term Performance Based on Elman Neural Network Model: Evidence from 2006 to 2019 in China. Complexity،Vol. 2020, no. 2020, pp.1-15.
https://search.emarefa.net/detail/BIM-1144572
Modern Language Association (MLA)
Xiao, Ming…[et al.]. M&A Short-Term Performance Based on Elman Neural Network Model: Evidence from 2006 to 2019 in China. Complexity No. 2020 (2020), pp.1-15.
https://search.emarefa.net/detail/BIM-1144572
American Medical Association (AMA)
Xiao, Ming& Yang, Xionghui& Li, Ge. M&A Short-Term Performance Based on Elman Neural Network Model: Evidence from 2006 to 2019 in China. Complexity. 2020. Vol. 2020, no. 2020, pp.1-15.
https://search.emarefa.net/detail/BIM-1144572
Data Type
Journal Articles
Language
English
Notes
Includes bibliographical references
Record ID
BIM-1144572