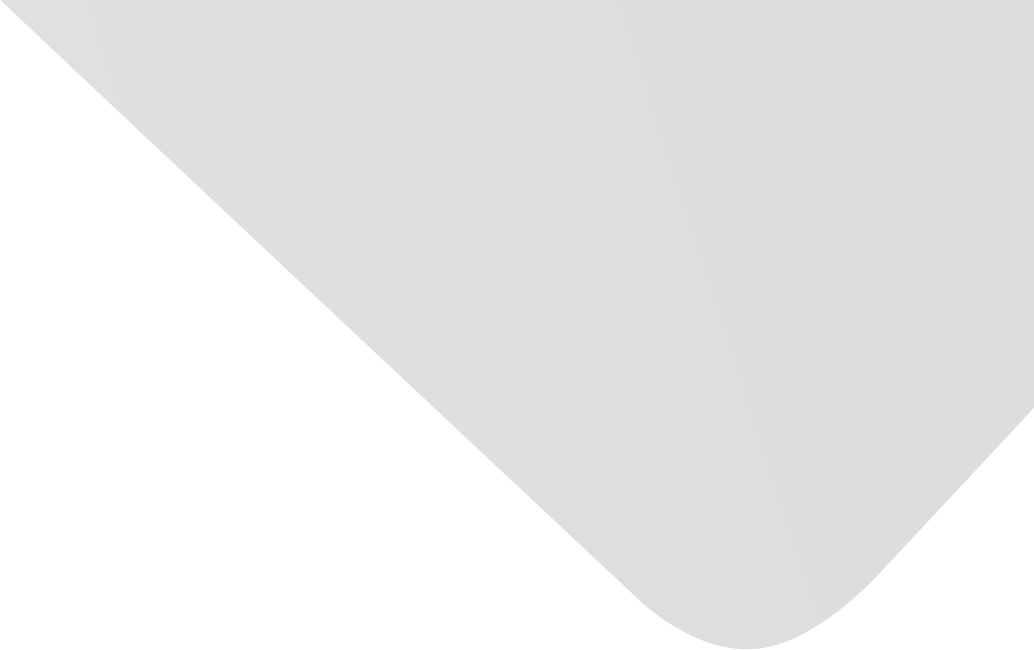
Prescribed Performance Neural Control of Strict-Feedback Systems via Disturbance Observers
Joint Authors
Yang, Chunzhi
Zhu, Fang
Xu, Guangkui
Xiang, Wei
Source
Issue
Vol. 2020, Issue 2020 (31 Dec. 2020), pp.1-12, 12 p.
Publisher
Hindawi Publishing Corporation
Publication Date
2020-10-29
Country of Publication
Egypt
No. of Pages
12
Main Subjects
Abstract EN
This paper provides a disturbance observer-based prescribed performance control method for uncertain strict-feedback systems.
To guarantee that the tracking error meets a design prescribed performance boundary (PPB) condition, an improved prescribed performance function is introduced.
And radial basis function neural networks (RBFNNs) are used to approximate nonlinear functions, while second-order filters are employed to eliminate the “explosion-complexity” problem inherent in the existing method.
Meanwhile, disturbance observers are constructed to estimate the compounded disturbance which includes time-varying disturbances and network construction errors.
The stability of the whole closed-loop system is guaranteed via Lyapunov theory.
Finally, comparative simulation results confirm that the proposed control method can achieve better tracking performance.
American Psychological Association (APA)
Xiang, Wei& Xu, Guangkui& Zhu, Fang& Yang, Chunzhi. 2020. Prescribed Performance Neural Control of Strict-Feedback Systems via Disturbance Observers. Complexity،Vol. 2020, no. 2020, pp.1-12.
https://search.emarefa.net/detail/BIM-1144772
Modern Language Association (MLA)
Xiang, Wei…[et al.]. Prescribed Performance Neural Control of Strict-Feedback Systems via Disturbance Observers. Complexity No. 2020 (2020), pp.1-12.
https://search.emarefa.net/detail/BIM-1144772
American Medical Association (AMA)
Xiang, Wei& Xu, Guangkui& Zhu, Fang& Yang, Chunzhi. Prescribed Performance Neural Control of Strict-Feedback Systems via Disturbance Observers. Complexity. 2020. Vol. 2020, no. 2020, pp.1-12.
https://search.emarefa.net/detail/BIM-1144772
Data Type
Journal Articles
Language
English
Notes
Includes bibliographical references
Record ID
BIM-1144772