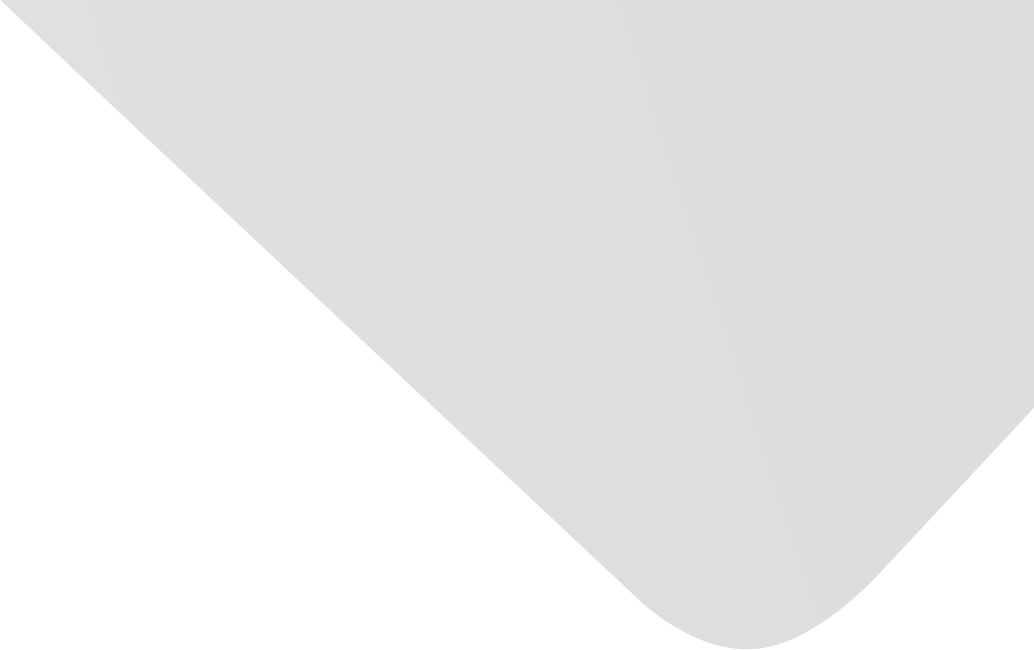
Bio-Inspired Structure Representation Based Cross-View Discriminative Subspace Learning via Simultaneous Local and Global Alignment
Joint Authors
Li, Ao
Ding, Yu
Zheng, Xunjiang
Chen, Deyun
Sun, Guanglu
Lin, Kezheng
Source
Issue
Vol. 2020, Issue 2020 (31 Dec. 2020), pp.1-14, 14 p.
Publisher
Hindawi Publishing Corporation
Publication Date
2020-11-04
Country of Publication
Egypt
No. of Pages
14
Main Subjects
Abstract EN
Recently, cross-view feature learning has been a hot topic in machine learning due to the wide applications of multiview data.
Nevertheless, the distribution discrepancy between cross-views leads to the fact that instances of the different views from same class are farther than those within the same view but from different classes.
To address this problem, in this paper, we develop a novel cross-view discriminative feature subspace learning method inspired by layered visual perception from human.
Firstly, the proposed method utilizes a separable low-rank self-representation model to disentangle the class and view structure layers, respectively.
Secondly, a local alignment is constructed with two designed graphs to guide the subspace decomposition in a pairwise way.
Finally, the global discriminative constraint on distribution center in each view is designed for further alignment improvement.
Extensive cross-view classification experiments on several public datasets prove that our proposed method is more effective than other existing feature learning methods.
American Psychological Association (APA)
Li, Ao& Ding, Yu& Zheng, Xunjiang& Chen, Deyun& Sun, Guanglu& Lin, Kezheng. 2020. Bio-Inspired Structure Representation Based Cross-View Discriminative Subspace Learning via Simultaneous Local and Global Alignment. Complexity،Vol. 2020, no. 2020, pp.1-14.
https://search.emarefa.net/detail/BIM-1145063
Modern Language Association (MLA)
Li, Ao…[et al.]. Bio-Inspired Structure Representation Based Cross-View Discriminative Subspace Learning via Simultaneous Local and Global Alignment. Complexity No. 2020 (2020), pp.1-14.
https://search.emarefa.net/detail/BIM-1145063
American Medical Association (AMA)
Li, Ao& Ding, Yu& Zheng, Xunjiang& Chen, Deyun& Sun, Guanglu& Lin, Kezheng. Bio-Inspired Structure Representation Based Cross-View Discriminative Subspace Learning via Simultaneous Local and Global Alignment. Complexity. 2020. Vol. 2020, no. 2020, pp.1-14.
https://search.emarefa.net/detail/BIM-1145063
Data Type
Journal Articles
Language
English
Notes
Includes bibliographical references
Record ID
BIM-1145063