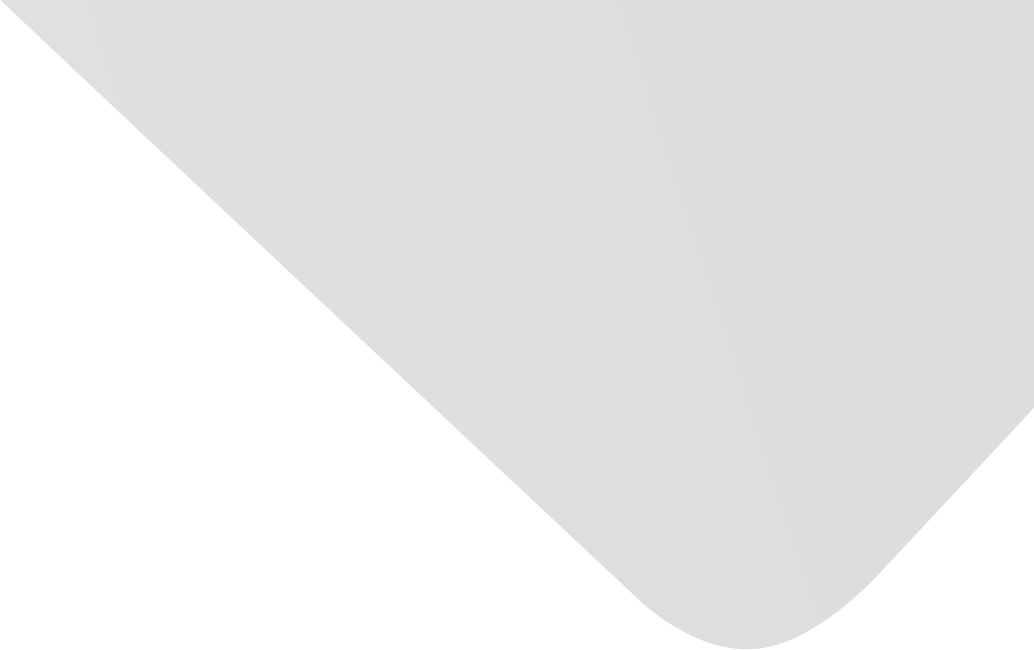
Optimal Skipping Rates: Training Agents with Fine-Grained Control Using Deep Reinforcement Learning
Joint Authors
Jiang, Feng
Khan, Adil
Liu, Shaohui
Asghar, Muhammad Zubair
Source
Issue
Vol. 2019, Issue 2019 (31 Dec. 2019), pp.1-10, 10 p.
Publisher
Hindawi Publishing Corporation
Publication Date
2019-03-03
Country of Publication
Egypt
No. of Pages
10
Main Subjects
Abstract EN
These days game AI is one of the focused and active research areas in artificial intelligence because computer games are the best test-beds for testing theoretical ideas in AI before practically applying them in real life world.
Similarly, ViZDoom is a game artificial intelligence research platform based on Doom used for visual deep reinforcement learning in 3D game environments such as first-person shooters (FPS).
While training, the speed of the learning agent greatly depends on the number of frames the agent is permitted to skip.
In this paper, how the frame skipping rate influences the agent’s learning and final performance is proposed, particularly using deep Q-learning, experience replay memory, and the ViZDoom Game AI research platform.
The agent is trained and tested on Doom’s basic scenario(s) where the results are compared and found to be 10% better compared to the existing state-of-the-art research work on Doom-based agents.
The experiments show that the profitable and optimal frame skipping rate falls in the range of 3 to 11 that provides the best balance between the learning speed and the final performance of the agent which exhibits human-like behavior and outperforms an average human player and inbuilt game agents.
American Psychological Association (APA)
Khan, Adil& Jiang, Feng& Liu, Shaohui& Asghar, Muhammad Zubair. 2019. Optimal Skipping Rates: Training Agents with Fine-Grained Control Using Deep Reinforcement Learning. Journal of Robotics،Vol. 2019, no. 2019, pp.1-10.
https://search.emarefa.net/detail/BIM-1186930
Modern Language Association (MLA)
Khan, Adil…[et al.]. Optimal Skipping Rates: Training Agents with Fine-Grained Control Using Deep Reinforcement Learning. Journal of Robotics No. 2019 (2019), pp.1-10.
https://search.emarefa.net/detail/BIM-1186930
American Medical Association (AMA)
Khan, Adil& Jiang, Feng& Liu, Shaohui& Asghar, Muhammad Zubair. Optimal Skipping Rates: Training Agents with Fine-Grained Control Using Deep Reinforcement Learning. Journal of Robotics. 2019. Vol. 2019, no. 2019, pp.1-10.
https://search.emarefa.net/detail/BIM-1186930
Data Type
Journal Articles
Language
English
Notes
Includes bibliographical references
Record ID
BIM-1186930