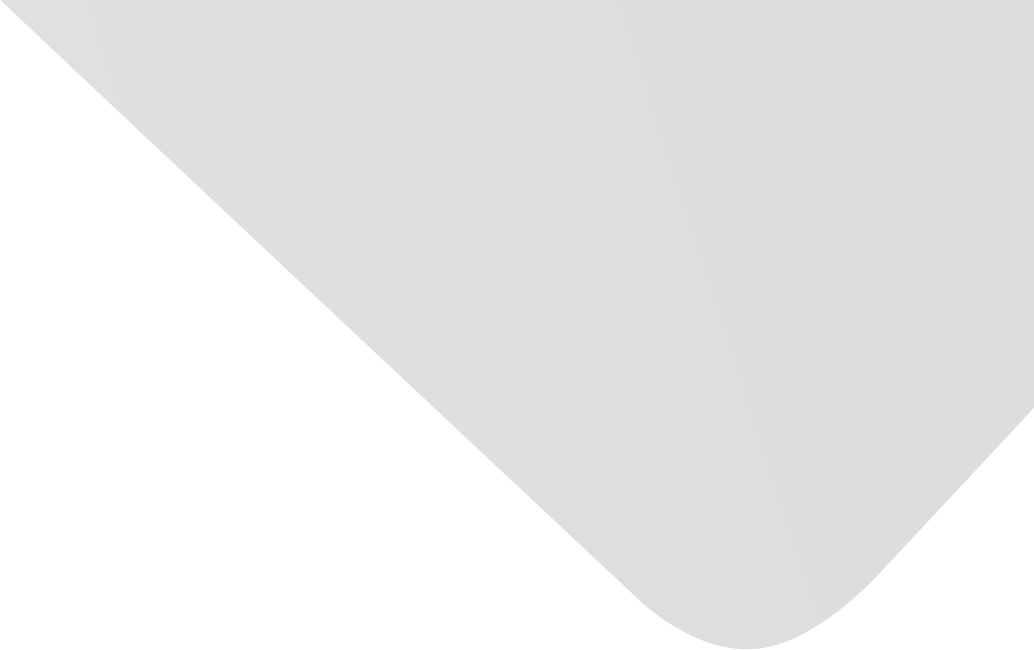
Learning from Demonstrations and Human Evaluative Feedbacks: Handling Sparsity and Imperfection Using Inverse Reinforcement Learning Approach
Joint Authors
Araabi, Babak N.
Nili Ahmadabadi, Majid
Mourad, Nafee
Ezzeddine, Ali
Source
Issue
Vol. 2020, Issue 2020 (31 Dec. 2020), pp.1-18, 18 p.
Publisher
Hindawi Publishing Corporation
Publication Date
2020-01-13
Country of Publication
Egypt
No. of Pages
18
Main Subjects
Abstract EN
Programming by demonstrations is one of the most efficient methods for knowledge transfer to develop advanced learning systems, provided that teachers deliver abundant and correct demonstrations, and learners correctly perceive them.
Nevertheless, demonstrations are sparse and inaccurate in almost all real-world problems.
Complementary information is needed to compensate these shortcomings of demonstrations.
In this paper, we target programming by a combination of nonoptimal and sparse demonstrations and a limited number of binary evaluative feedbacks, where the learner uses its own evaluated experiences as new demonstrations in an extended inverse reinforcement learning method.
This provides the learner with a broader generalization and less regret as well as robustness in face of sparsity and nonoptimality in demonstrations and feedbacks.
Our method alleviates the unrealistic burden on teachers to provide optimal and abundant demonstrations.
Employing an evaluative feedback, which is easy for teachers to deliver, provides the opportunity to correct the learner’s behavior in an interactive social setting without requiring teachers to know and use their own accurate reward function.
Here, we enhance the inverse reinforcement learning (IRL) to estimate the reward function using a mixture of nonoptimal and sparse demonstrations and evaluative feedbacks.
Our method, called IRL from demonstration and human’s critique (IRLDC), has two phases.
The teacher first provides some demonstrations for the learner to initialize its policy.
Next, the learner interacts with the environment and the teacher provides binary evaluative feedbacks.
Taking into account possible inconsistencies and mistakes in issuing and receiving feedbacks, the learner revises the estimated reward function by solving a single optimization problem.
The IRLDC is devised to handle errors and sparsities in demonstrations and feedbacks and can generalize different combinations of these two sources expertise.
We apply our method to three domains: a simulated navigation task, a simulated car driving problem with human interactions, and a navigation experiment of a mobile robot.
The results indicate that the IRLDC significantly enhances the learning process where the standard IRL methods fail and learning from feedbacks (LfF) methods has a high regret.
Also, the IRLDC works well at different levels of sparsity and optimality of the teacher’s demonstrations and feedbacks, where other state-of-the-art methods fail.
American Psychological Association (APA)
Mourad, Nafee& Ezzeddine, Ali& Araabi, Babak N.& Nili Ahmadabadi, Majid. 2020. Learning from Demonstrations and Human Evaluative Feedbacks: Handling Sparsity and Imperfection Using Inverse Reinforcement Learning Approach. Journal of Robotics،Vol. 2020, no. 2020, pp.1-18.
https://search.emarefa.net/detail/BIM-1190218
Modern Language Association (MLA)
Mourad, Nafee…[et al.]. Learning from Demonstrations and Human Evaluative Feedbacks: Handling Sparsity and Imperfection Using Inverse Reinforcement Learning Approach. Journal of Robotics No. 2020 (2020), pp.1-18.
https://search.emarefa.net/detail/BIM-1190218
American Medical Association (AMA)
Mourad, Nafee& Ezzeddine, Ali& Araabi, Babak N.& Nili Ahmadabadi, Majid. Learning from Demonstrations and Human Evaluative Feedbacks: Handling Sparsity and Imperfection Using Inverse Reinforcement Learning Approach. Journal of Robotics. 2020. Vol. 2020, no. 2020, pp.1-18.
https://search.emarefa.net/detail/BIM-1190218
Data Type
Journal Articles
Language
English
Notes
Includes bibliographical references
Record ID
BIM-1190218