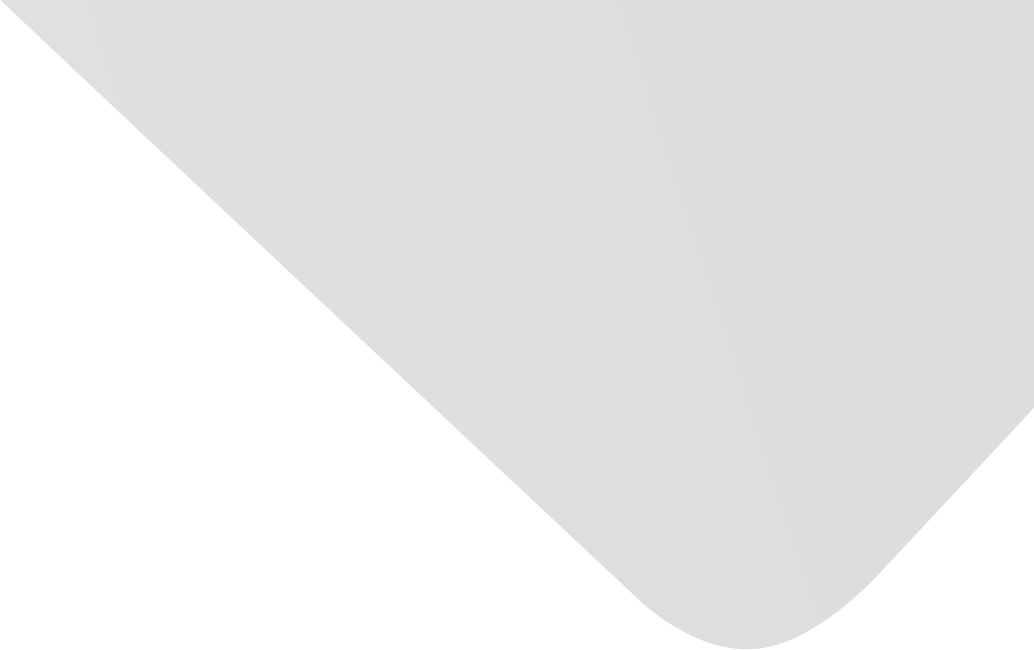
A Multiple Hidden Layers Extreme Learning Machine Method and Its Application
Joint Authors
Mao, Yachun
Li, Beijing
Xiao, Dong
Source
Mathematical Problems in Engineering
Issue
Vol. 2017, Issue 2017 (31 Dec. 2017), pp.1-10, 10 p.
Publisher
Hindawi Publishing Corporation
Publication Date
2017-12-13
Country of Publication
Egypt
No. of Pages
10
Main Subjects
Abstract EN
Extreme learning machine (ELM) is a rapid learning algorithm of the single-hidden-layer feedforward neural network, which randomly initializes the weights between the input layer and the hidden layer and the bias of hidden layer neurons and finally uses the least-squares method to calculate the weights between the hidden layer and the output layer.
This paper proposes a multiple hidden layers ELM (MELM for short) which inherits the characteristics of parameters of the first hidden layer.
The parameters of the remaining hidden layers are obtained by introducing a method (make the actual output zero error approach the expected hidden layer output).
Based on the MELM algorithm, many experiments on regression and classification show that the MELM can achieve the satisfactory results based on average precision and good generalization performance compared to the two-hidden-layer ELM (TELM), the ELM, and some other multilayer ELM.
American Psychological Association (APA)
Xiao, Dong& Li, Beijing& Mao, Yachun. 2017. A Multiple Hidden Layers Extreme Learning Machine Method and Its Application. Mathematical Problems in Engineering،Vol. 2017, no. 2017, pp.1-10.
https://search.emarefa.net/detail/BIM-1190466
Modern Language Association (MLA)
Xiao, Dong…[et al.]. A Multiple Hidden Layers Extreme Learning Machine Method and Its Application. Mathematical Problems in Engineering No. 2017 (2017), pp.1-10.
https://search.emarefa.net/detail/BIM-1190466
American Medical Association (AMA)
Xiao, Dong& Li, Beijing& Mao, Yachun. A Multiple Hidden Layers Extreme Learning Machine Method and Its Application. Mathematical Problems in Engineering. 2017. Vol. 2017, no. 2017, pp.1-10.
https://search.emarefa.net/detail/BIM-1190466
Data Type
Journal Articles
Language
English
Notes
Includes bibliographical references
Record ID
BIM-1190466