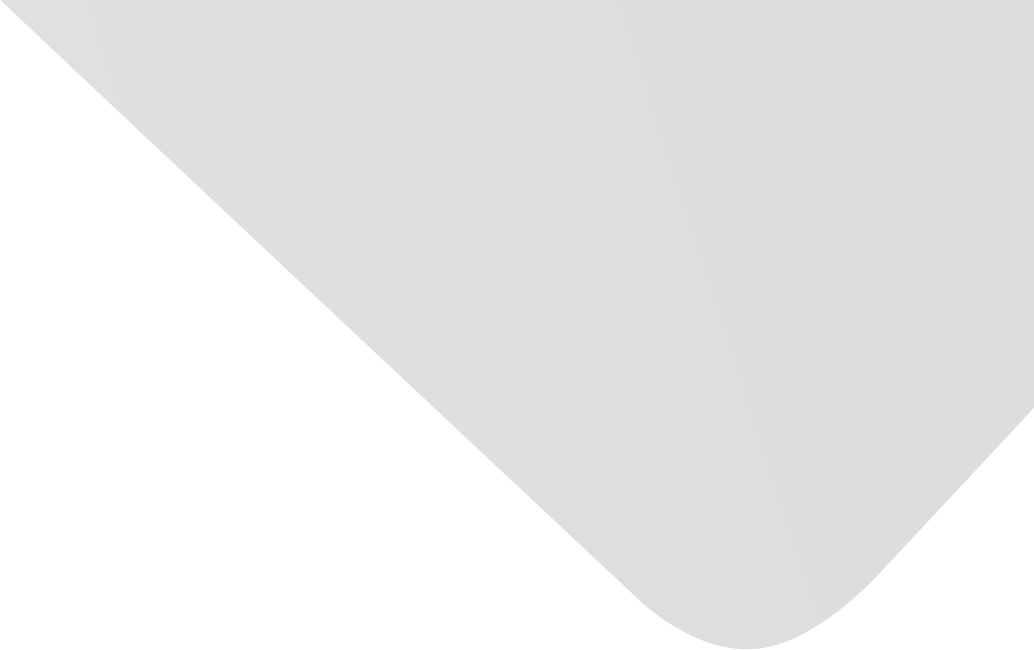
Semantic segmentation of educational videos for micro learning objects in adaptive e-learning
Other Title(s)
التقسيم الدلالي لمقاطع الفيديو التعليمية لكائنات التعلم الصغيرة في التعلم الإلكتروني التكيفي
Joint Authors
Nasr, Abd al-Rahman Ali
Jamal al-Din, Shihab
Halawah, Abd al-Rahman Samir
Source
Journal of Al-Azhar University Engineering Sector
Issue
Vol. 18, Issue 69 (31 Oct. 2023), pp.900-913, 14 p.
Publisher
al-Azhar University Faculty of Engineering
Publication Date
2023-10-31
Country of Publication
Egypt
No. of Pages
14
Main Subjects
Information Technology and Computer Science
Topics
Abstract AR
التعلم الإلكتروني يكتسب شهرة، خاصة في التعلم طويل المدى، وذلك بشكل رئيسي من خلال المحاضرات التي تحتوي مقاطع الفيديو.
ومع ذلك، تغطي هذه المقاطع في كثير من الأحيان مواضيع متعددة أو تخدم أدوارا تعليمية متنوعة ضمن موضوع واحد في التعلم الإلكتروني التكيفي، كلما كانت الوحدات أصغر وأكثر تفصيلا، كلما تم إعداد عروض ومحاضرات مخصصة أكثر مرونة.
تعرف مثل هذه الوحدات بأسماء كائنات التعلم الصغيرة Micro Learning Objects (MLOs).
وبناء على ذلك، يظهر الحاجة إلى تقسيم مقاطع فيديو المحاضرات هذه إلى MLOs متعددة، حيث يقوم كل منها بأداء دور تعليمي مميز في المحاضرة يقدم هذا المقال نموذجا تلقائيا يستفيد من نماذج اللغة المتقدمة لتقسيم مقاطع فيديو المحاضرات بشكل دلالي إلى كائنات تعلم صغيرة (MLOS) بالإضافة إلى ذلك، تم تقديم مجموعة بيانات جديدة مجزأة جيدا لمقاطع الفيديو التعليمية (YT-EV)، حيث يتم تقسيم الفيديو وفقا لجدول زمني محدد مسبقا.
تم تدريب النموذج على مجموعات بيانات نصية عامة لفهم أقسام كائنات التعلم ومن ثم يتم ضبطه بشكل أفضل باستخدام التعلم عبر النقل على مجموعات بيانات الفيديو لتحقيق نتائج تقسيم أفضل.
أظهرت النتائج التجريبية F1-score بقيمة 0.657، والتي تعتبر واعدة وتؤكد على أهمية تجزئة الفيديو المستندة إلى النص لتعزيز التعلم الإلكتروني التكيفي.
Abstract EN
E-Learning is gaining prominence, especially in lifelong learning, primarily through lecture videos.
however, these videos often encompass multiple topics or serve various instructional roles within a single subject.
in adaptive e-learning, the smaller and granular the units, the more versatile presentations and personalized lectures are composed.
such units are known as micro learning objects (MLOS).
consequently, the necessity emerges to segment these lecture videos into multiple MLOS, each fulfilling a distinct instructional role in a lecture.
this article presents an automatic model leveraging advanced language models to segment lecture videos semantically into micro learning objects (MLOS).
additionally, a new well-segmented dataset of educational videos (Yt-Ev) was introduced, in which the video is segmented according to a pre-defined timestamped agenda.
the model is trained on general text datasets to understand lo segments and subsequently fine-tuned using transfer learning on video datasets to achieve better segmentation results.
the experimental results showed an F1-score of value 0.657, which is considered promising and emphasizes the significance of text transcript-based video segmentation for enhancing adaptive E-learning.
American Psychological Association (APA)
Halawah, Abd al-Rahman Samir& Jamal al-Din, Shihab& Nasr, Abd al-Rahman Ali. 2023. Semantic segmentation of educational videos for micro learning objects in adaptive e-learning. Journal of Al-Azhar University Engineering Sector،Vol. 18, no. 69, pp.900-913.
https://search.emarefa.net/detail/BIM-1519782
Modern Language Association (MLA)
Halawah, Abd al-Rahman Samir…[et al.]. Semantic segmentation of educational videos for micro learning objects in adaptive e-learning. Journal of Al-Azhar University Engineering Sector Vol. 18, no. 69 (Oct. 2023), pp.900-913.
https://search.emarefa.net/detail/BIM-1519782
American Medical Association (AMA)
Halawah, Abd al-Rahman Samir& Jamal al-Din, Shihab& Nasr, Abd al-Rahman Ali. Semantic segmentation of educational videos for micro learning objects in adaptive e-learning. Journal of Al-Azhar University Engineering Sector. 2023. Vol. 18, no. 69, pp.900-913.
https://search.emarefa.net/detail/BIM-1519782
Data Type
Journal Articles
Language
English
Notes
Includes bibliographical references: p. 911-913
Record ID
BIM-1519782