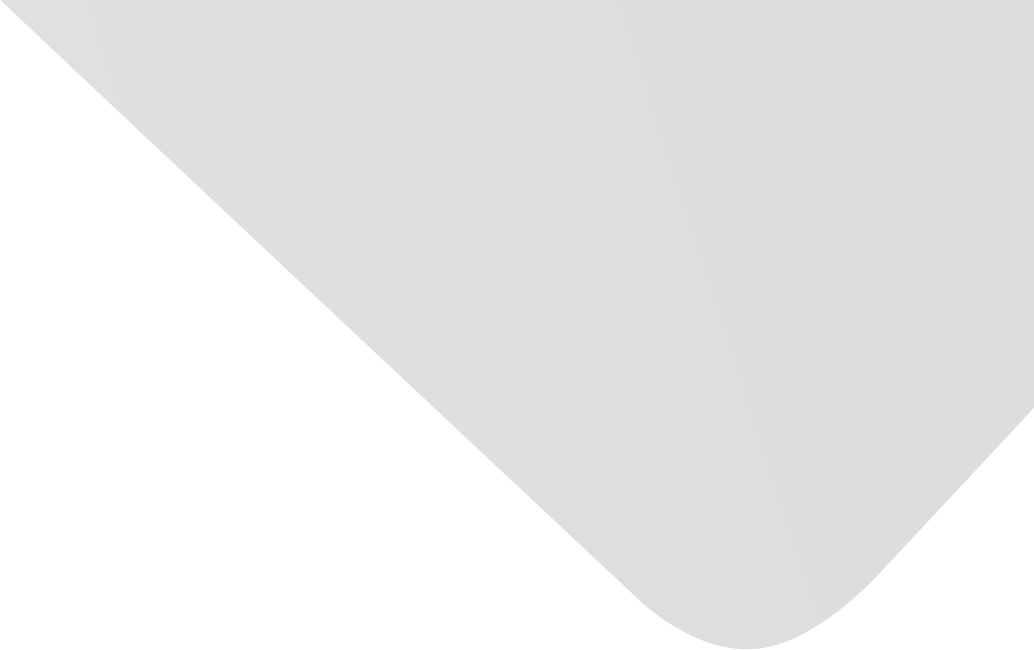
Seasonal Egyptian road traffic volume variations using machine learning
Other Title(s)
التغيرات الموسمية في حجم حركة المرور على الطرق المصرية باستخدام التعلم الآلي
Joint Authors
Ramadan, Ibrahim Muhammad
Abd al-Qadir, Taha Izzat
Kamal, Sharif Ahmad
Source
Journal of Al-Azhar University Engineering Sector
Issue
Vol. 19, Issue 71 (30 Apr. 2024), pp.475-494, 20 p.
Publisher
al-Azhar University Faculty of Engineering
Publication Date
2024-04-30
Country of Publication
Egypt
No. of Pages
20
Main Subjects
Abstract AR
يؤثر الطقس والإجازات والجداول المدرسية على العوامل الموسمية (ST) وبالتالي تتأثر قيم متوسط حركة المرور اليومية السنوية AADT وحركة المرور المستقبلية، وعليه يجب أن تتضمن بيانات AADT الخصائص الموسمية لفهم تدفق حركة المرور والتخطيط الجيد للنقل.
تحسن هذه الدراسة تقدير العوامل الموسمية (SI) في مصر حيث لم يكن لدى الهيئة العامة للطرق والكباري GARBLT بيانات الحصر حركة المرور منذ عام 2018 والذي تسبب في العديد من المشكلات المرورية.
تم استخدام البيانات الخاصة بمحطات الرصد المستمرة لعدد 11 محطة رصد ثابتة (التابعة للهيئة العامة للطرق والكباري) في الفترة من عام 2013 إلى 2018 بالإضافة إلى البيانات الاجتماعية والاقتصادية القائمة على خصائص الطريق مثل عدد ممرات الطرق وموقع المحطة، تم حساب قيمة AADT بشكل أكثر دقة وسهولة بعد الحصول على العوامل الموسمية.
تنبأت الشبكات العصبية بالعوامل الموسمية بشكل أفضل من طريقة الانحدار.
باستخدام قيم ST، يمكن حساب حجم حركة المرور للطرق المتعددة من فترة مراقبة قصيرة، ومن خلال الدراسة يمكن التخطيط الجيد لوسائل النقل المستقبلية للتعامل مع أكبر قضايا النقل في مصر.
Abstract EN
Seasonal factors weather, holidays, and school schedules all contribute to traffic.
seasonal factors have a significant influence on annual average daily traffic (AADT).
traffic volume is measured and predicted using AADT.
to make intelligent transportation planning and finance decisions, seasonal factors must be included while examining AADT data since there has been no Egyptian traffic flow research.
machine learning approaches include regression analysis and artificial neural networks (ANNS) to predict seasonal variations.
since 2018, the general authority for roads, bridges, and land transport (GARBLT) has not kept traffic records.
seasonal factors were employed to generate a more accurate and understandable AADT figure from 11 stationary monitoring stations monitored from 2013 to 2018.
these stations collected socioeconomic data as well as information about the roads, such as lanes and station locations.
the artificial neural network (ANN) model seasonal factors were far more precise and reliable when compared to the real values.
American Psychological Association (APA)
Ramadan, Ibrahim Muhammad& Abd al-Qadir, Taha Izzat& Kamal, Sharif Ahmad. 2024. Seasonal Egyptian road traffic volume variations using machine learning. Journal of Al-Azhar University Engineering Sector،Vol. 19, no. 71, pp.475-494.
https://search.emarefa.net/detail/BIM-1563426
Modern Language Association (MLA)
Ramadan, Ibrahim Muhammad…[et al.]. Seasonal Egyptian road traffic volume variations using machine learning. Journal of Al-Azhar University Engineering Sector Vol. 19, no. 71 (Apr. 2024), pp.475-494.
https://search.emarefa.net/detail/BIM-1563426
American Medical Association (AMA)
Ramadan, Ibrahim Muhammad& Abd al-Qadir, Taha Izzat& Kamal, Sharif Ahmad. Seasonal Egyptian road traffic volume variations using machine learning. Journal of Al-Azhar University Engineering Sector. 2024. Vol. 19, no. 71, pp.475-494.
https://search.emarefa.net/detail/BIM-1563426
Data Type
Journal Articles
Language
English
Notes
Includes bibliographical references: p. 491-494
Record ID
BIM-1563426