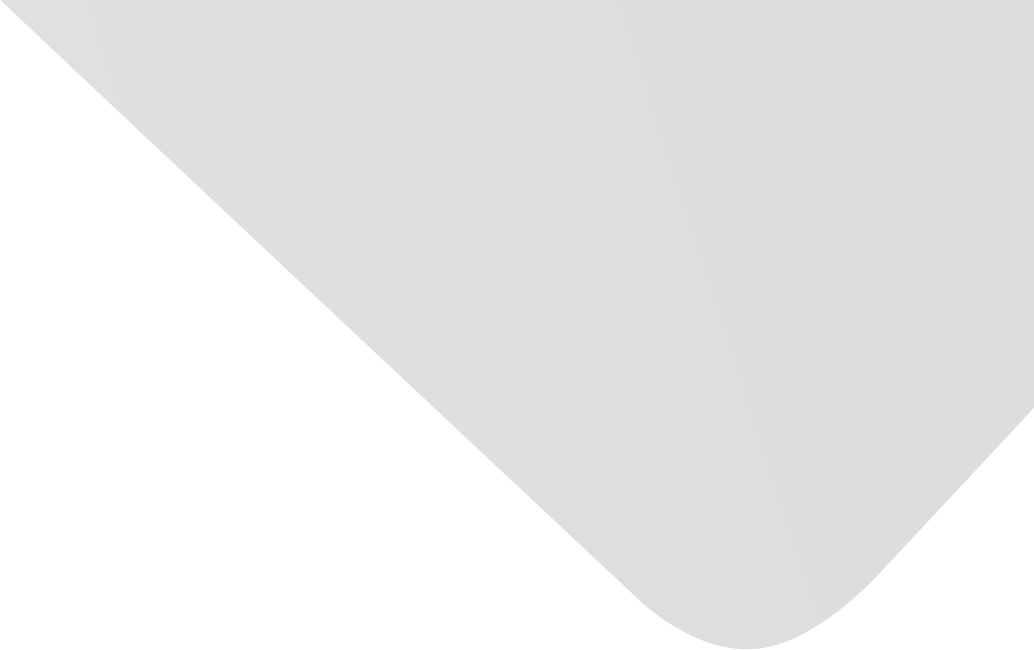
Evaluation of data mining classification models
Other Title(s)
تقييم نماذج مصنفات التنقيب عن البيانات
Joint Authors
al-Habi, Abd Allah M.
al-Gharib, Muhammad
Source
IUG Journal of Natural Studies
Issue
Vol. 22, Issue 1 (30 Jun. 2014), pp.151-165, 15 p.
Publisher
The Islamic University-Gaza Deanship of Research and Graduate Affairs
Publication Date
2014-06-30
Country of Publication
Palestine (Gaza Strip)
No. of Pages
15
Main Subjects
Information Technology and Computer Science
Topics
Abstract AR
تهدف هذه الورقة البحثية إلى التعرف على أشهر تقنيات التنقيب عن البيانات و تقييمها، عادة ما تسمى هذه التقنيات بالمصنفات، و ذلك لاستخدامها في تصنيف المتغيرات التابعة الوصفية أو الفئوية.
حيث تم تقييم خمس من هذه المصنفات و هي : شجرة القرار، و الشبكات العصبية، و آلة دعم المتجه، و مصنف بيز، و الجار الأقرب من خلال قياس فعالية هذه المصنفات و الحصول منها على نماذج تنبؤية للتصنيف، تم عمل دراسة محاكاة و تبين منها أن الشبكات العصبية، و آلة دعم المتجه، و الجار الأقرب أعطت نتائج عالية نوعا ما في عملية التصنيف، و كذلك تم في هذه الدراسة تطبيق هذه المصنفات على ثلاث مجموعات مختلفة من البيانات من حيث الحجم، فمنها الكبيرة نسبيا و منها متوسطة الحجم، و كانت هذه البيانات مختلفة أيضا من حيث نوع المتغيرات المستقلة (كمية، أو ترتيبية، أو وصفية)، و أيضا من حيث عدد فئات المتغير التابع)، (ثنائية الفئة، متعددة الفئات).
و لتقدير دقة التصنيف لهذه النماذج قد تم تطبيق هذه المصنفات على طريقتان من طرق قياس الفعالية و هي : Hold out validation و 10-Fold Cross validation و كانت المعيار الرئيسي في تقييم هذه المصنفات و النماذج التنبؤية هو التقييم من حيث الدقة الكلية في التصنيف.
تبين لنا من هذه الدراسة وجود بعض الاختلافات بين هذه المصنفات في دقة تصنيفها للمتغير التابع ثنائي الفئة في مجموعة البيانات الكبيرة نسبيا، و تصنيف المتغير التابع ذو الثلاث فئات في مجموعة البيانات المتوسطة الحجم، و أيضا في تصنيف المتغير التابع ذو السبع فئات في مجموعة البيانات المتوسطة الحجم عند استخدامنا كل من Hold out validation و validation 10-Fold Cross لقياس فعالية هذه المصنفات.
كما تبين أن نموذج آلة دعم المتجه هو الأفضل من بين هذه المصنفات المتنافسة، حيث أظهر أعلى دقة في تصنيف تلك المتغيرات باستخدام كلا الطريقتان في قياس الفعالية كما أظهرت هذه الدراسة أن طريقة validation 10-Fold Cross قد زادت من فعالية و دقة هذه المصنفات و قد كانت النتائج في حالتي دراسة المحاكاة و البيانات الحقيقية متقاربة نوعا ما.
Abstract EN
This paper aims to identify and evaluate data mining algorithms which are commonly implemented in supervised classification task.
Decision tree, Neural networks, Support Vector Machines (SVM), Naive Bayes, and K-Nearest Neighbor classifiers are evaluated by conducting a simulation study and then assigned to three different datasets to classify and predict the class membership of binary (2-class) and multi-class categorical dependent variables present in these datasets, these datasets were different among each other regarding their size (relatively large and small), and type of predictors (ordinal, numeric, and categorical), as well as number of classes associated with the categorical dependent variable presents in each datasets.
Classification performance of these models obtained from a hold-out and 10-fold cross-validation, and empirically evaluated regarding to their overall classification accuracy.
We concluded that, there are some differences between the classifiers accuracies, validated by using Hold out and 10-fold cross validation methods assigned to classify a binary categorical dependent variable presents in relatively large dataset, a (3-class) categorical dependent variable presents in relatively small dataset, and a (7-class) categorical dependent variable presents in relatively small dataset, SVM classifier gave the highest averaged rate of classification accuracy in the both methods of validation assigned to these different datasets.
Therefore, we can conclude that the SVM, Neural networks, and k-Nearest Neighbor gave the highest averaged rate of classification, and 10-fold cross validation increased the classifiers accuracies.
And this result is approximately matching the conducted simulation results.
American Psychological Association (APA)
al-Habi, Abd Allah M.& al-Gharib, Muhammad. 2014. Evaluation of data mining classification models. IUG Journal of Natural Studies،Vol. 22, no. 1, pp.151-165.
https://search.emarefa.net/detail/BIM-354700
Modern Language Association (MLA)
al-Habi, Abd Allah M.& al-Gharib, Muhammad. Evaluation of data mining classification models. IUG Journal of Natural Studies Vol. 22, no. 1 (2014), pp.151-165.
https://search.emarefa.net/detail/BIM-354700
American Medical Association (AMA)
al-Habi, Abd Allah M.& al-Gharib, Muhammad. Evaluation of data mining classification models. IUG Journal of Natural Studies. 2014. Vol. 22, no. 1, pp.151-165.
https://search.emarefa.net/detail/BIM-354700
Data Type
Journal Articles
Language
English
Notes
Includes bibliographical references : p. 164-165
Record ID
BIM-354700