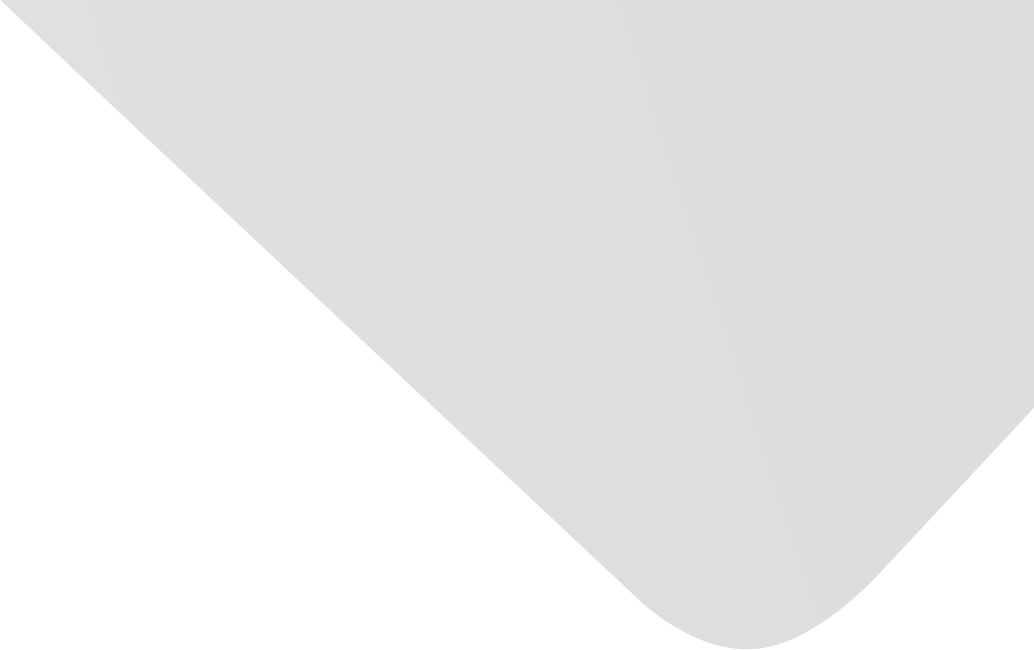
Kernel Sliced Inverse Regression : Regularization and Consistency
Joint Authors
Liang, Feng
Mukherjee, Sayan
Wu, Qiang
Source
Issue
Vol. 2013, Issue 2013 (31 Dec. 2013), pp.1-11, 11 p.
Publisher
Hindawi Publishing Corporation
Publication Date
2013-07-17
Country of Publication
Egypt
No. of Pages
11
Main Subjects
Abstract EN
Kernel sliced inverse regression (KSIR) is a natural framework for nonlinear dimension reduction using the mapping induced by kernels.
However, there are numeric, algorithmic, and conceptual subtleties in making the method robust and consistent.
We apply two types of regularization in this framework to address computational stability and generalization performance.
We also provide an interpretation of the algorithm and prove consistency.
The utility of this approach is illustrated on simulated and real data.
American Psychological Association (APA)
Wu, Qiang& Liang, Feng& Mukherjee, Sayan. 2013. Kernel Sliced Inverse Regression : Regularization and Consistency. Abstract and Applied Analysis،Vol. 2013, no. 2013, pp.1-11.
https://search.emarefa.net/detail/BIM-479917
Modern Language Association (MLA)
Wu, Qiang…[et al.]. Kernel Sliced Inverse Regression : Regularization and Consistency. Abstract and Applied Analysis No. 2013 (2013), pp.1-11.
https://search.emarefa.net/detail/BIM-479917
American Medical Association (AMA)
Wu, Qiang& Liang, Feng& Mukherjee, Sayan. Kernel Sliced Inverse Regression : Regularization and Consistency. Abstract and Applied Analysis. 2013. Vol. 2013, no. 2013, pp.1-11.
https://search.emarefa.net/detail/BIM-479917
Data Type
Journal Articles
Language
English
Notes
Includes bibliographical references
Record ID
BIM-479917