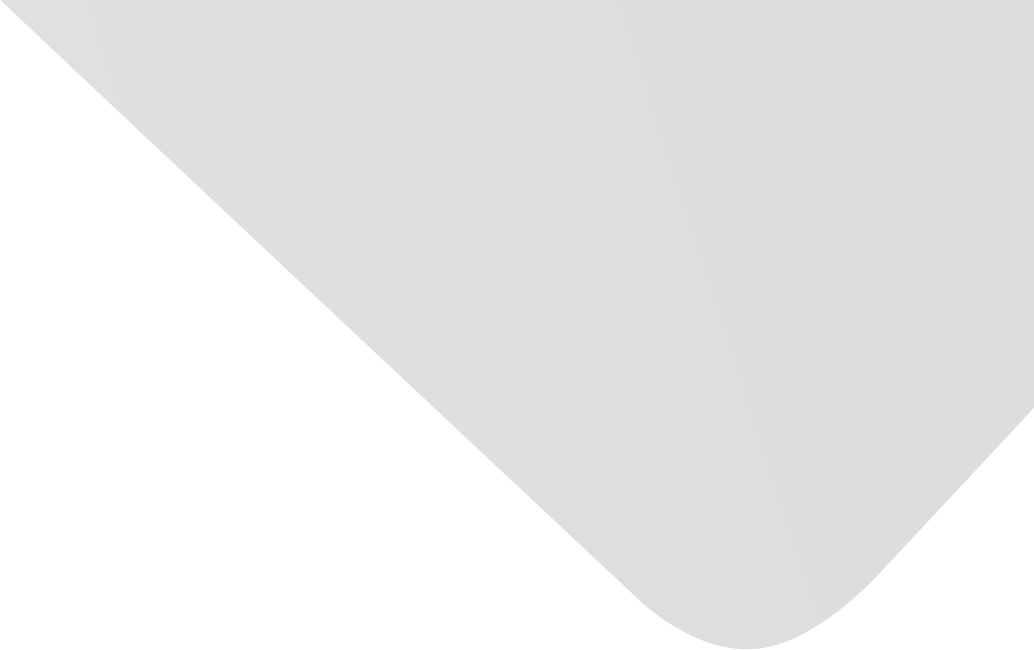
Convolutional Neural Network Applied to Traversability Analysis of Vehicles
Joint Authors
Wang, Mengmeng
Xinli, Ding
Linhui, Li
Lian, Jing
Yunpeng, Zong
Source
Advances in Mechanical Engineering
Issue
Vol. 2013, Issue 2013 (31 Dec. 2013), pp.1-6, 6 p.
Publisher
Hindawi Publishing Corporation
Publication Date
2013-11-12
Country of Publication
Egypt
No. of Pages
6
Main Subjects
Abstract EN
We focus on the need for traversability analysis of vehicles with convolutional neural networks.
Most related approaches to traversability analysis of vehicles suffer from the limitations imposed by extracting explicit features, algorithm scalability, and environment adaptivity.
In views of this, an approach based on the convolutional neural network (CNN) is presented to traversability analysis of vehicles, which can extract implicit features.
Besides, in order to enhance the training speed and accuracy, preprocessing and normalization are adopted before training.
The experimental results demonstrate that our method achieves high accuracy and strong robustness.
American Psychological Association (APA)
Linhui, Li& Wang, Mengmeng& Xinli, Ding& Lian, Jing& Yunpeng, Zong. 2013. Convolutional Neural Network Applied to Traversability Analysis of Vehicles. Advances in Mechanical Engineering،Vol. 2013, no. 2013, pp.1-6.
https://search.emarefa.net/detail/BIM-480136
Modern Language Association (MLA)
Linhui, Li…[et al.]. Convolutional Neural Network Applied to Traversability Analysis of Vehicles. Advances in Mechanical Engineering No. 2013 (2013), pp.1-6.
https://search.emarefa.net/detail/BIM-480136
American Medical Association (AMA)
Linhui, Li& Wang, Mengmeng& Xinli, Ding& Lian, Jing& Yunpeng, Zong. Convolutional Neural Network Applied to Traversability Analysis of Vehicles. Advances in Mechanical Engineering. 2013. Vol. 2013, no. 2013, pp.1-6.
https://search.emarefa.net/detail/BIM-480136
Data Type
Journal Articles
Language
English
Notes
Includes bibliographical references
Record ID
BIM-480136